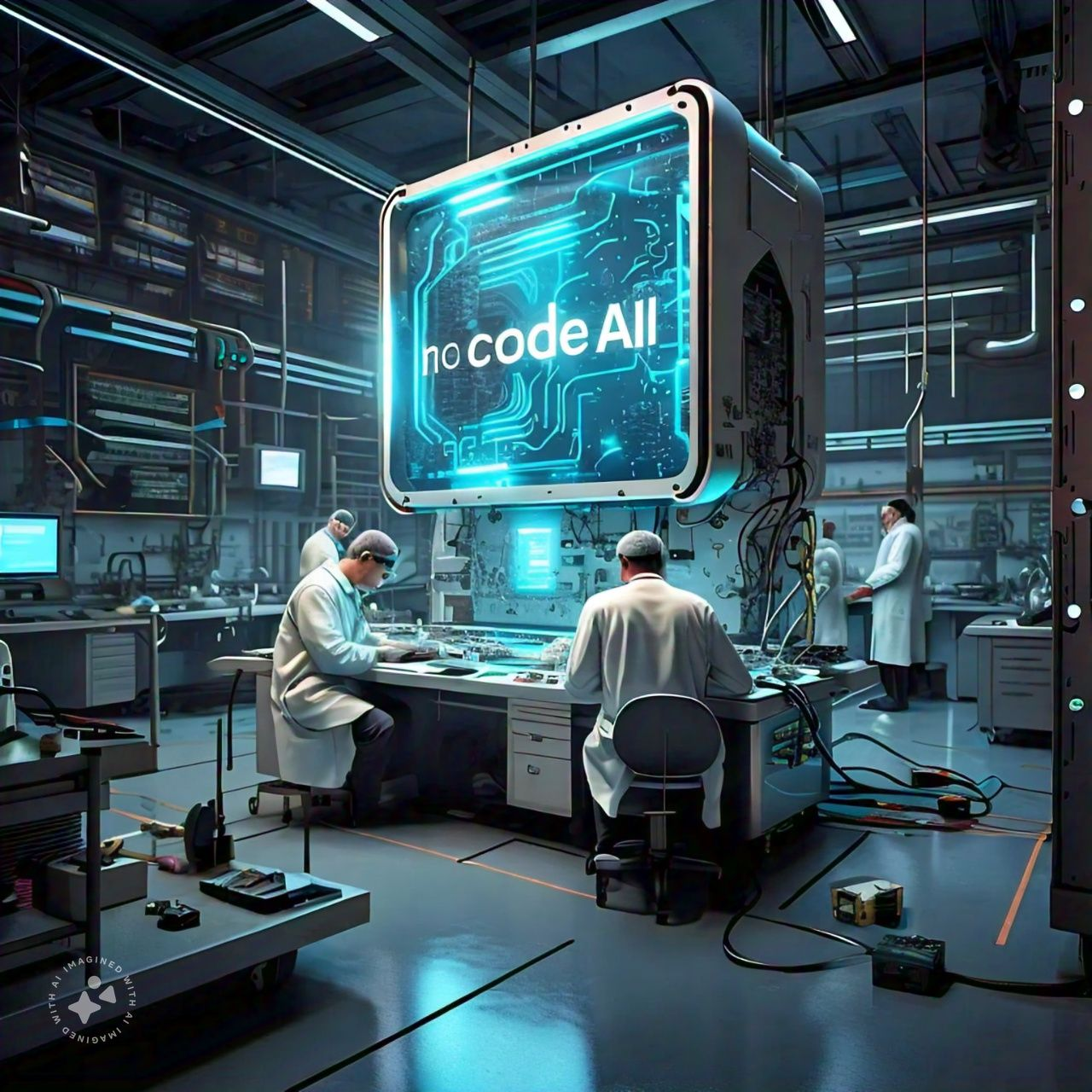
What is No-Code AI ?
No-code AI platforms are tools of empowerment, allowing users to build AI-driven applications through intuitive, drag-and-drop interfaces. These platforms typically offer pre-built modules for machine learning, data processing, and automation, eliminating the need for programming skills. Instead of writing code, users can connect the dots to create AI models that serve their specific needs. Whether you’re a marketer, entrepreneur, or developer, no-code AI makes the technology accessible to everyone, empowering you to create solutions that were once out of reach.
Artificial Intelligence (AI) has long been viewed as the domain of data scientists, requiring advanced coding skills and technical expertise. However, with the rise of no-code AI platforms, this is changing. Now, individuals and businesses can build robust AI solutions without writing a single line of code.
Benefits of No-Code AI
Accessibility
With no-code AI platforms, anyone can experiment with AI without needing a background in data science. This democratizes AI, allowing small businesses and individuals to harness its power without a hefty budget or a team of developers.
Speed
Traditional AI development can be a time-consuming process, often taking months from writing code to training models. No-code platforms significantly reduce this development time by offering pre-built templates and easy-to-use interfaces. A solution that may have taken months to develop can now be built in days, making your work more efficient and productive.
Cost-Effective
Hiring data scientists and developers to build custom AI solutions can be a significant expense. No-code AI platforms offer a more affordable alternative by providing tools at a fraction of the cost, making AI more accessible for small and medium-sized businesses. This cost-effectiveness can provide a sense of financial security and resourcefulness, knowing that you can harness the power of AI without breaking the bank.
Customization
Although these platforms are built for ease of use, they still offer flexibility in terms of customization. Users can tailor AI models to fit specific business needs, such as automating customer service responses, personalizing marketing efforts, or analyzing customer data for trends.
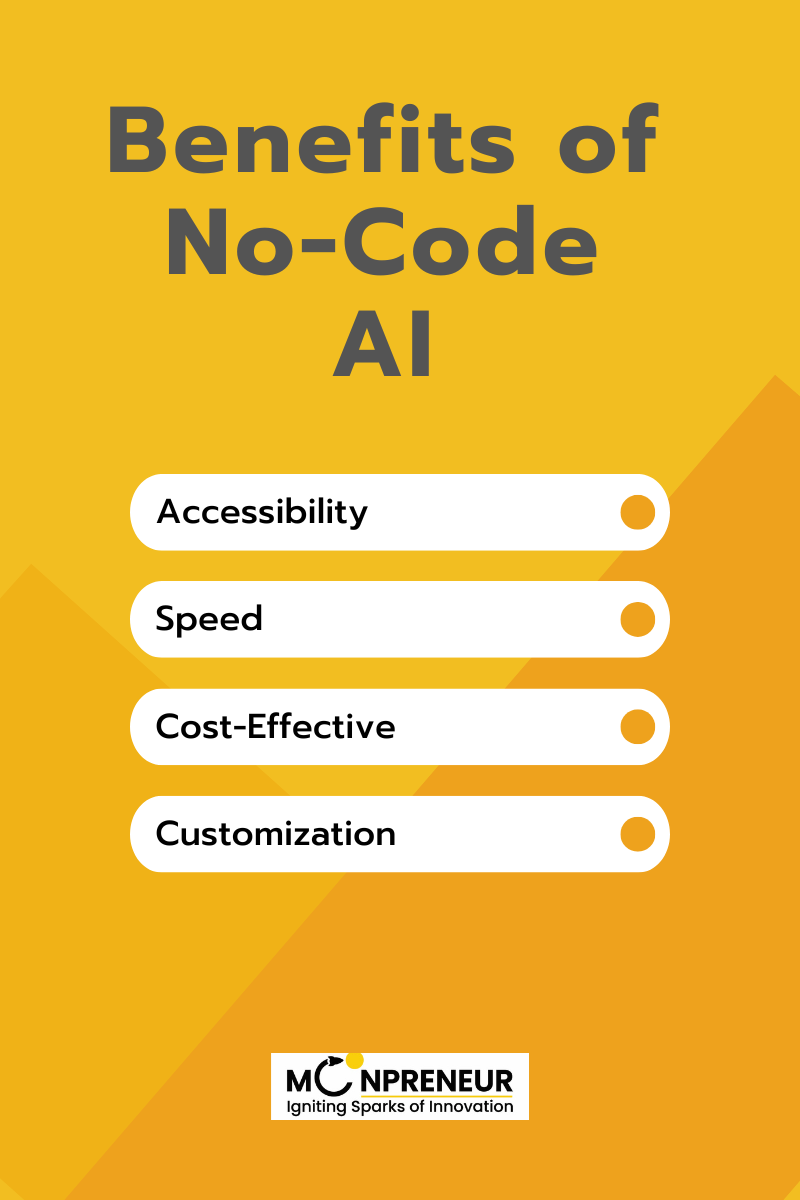
Popular No-Code AI Platforms
Google AutoML
Google’s AutoML stands out with its ability to build custom machine-learning models through an intuitive interface. The platform’s unique feature is its automatic selection of the best model for your data and its optimization for peak performance.
Teachable Machine
Teachable Machine by Google is an incredibly user-friendly platform for building image, sound, and pose-recognition models. It’s perfect for educators, content creators, and small businesses wanting to implement AI quickly.
Lobe
Lobe, owned by Microsoft, allows you to build custom AI models by dragging and dropping labeled images into the platform. It automatically trains your model without requiring any code.
MonkeyLearn
MonkeyLearn, a text analysis specialist, offers a relief from manual tasks by automating customer support and sentiment analysis. Its intuitive interface allows users to build machine-learning models for various text-based tasks, like email classification and opinion mining.
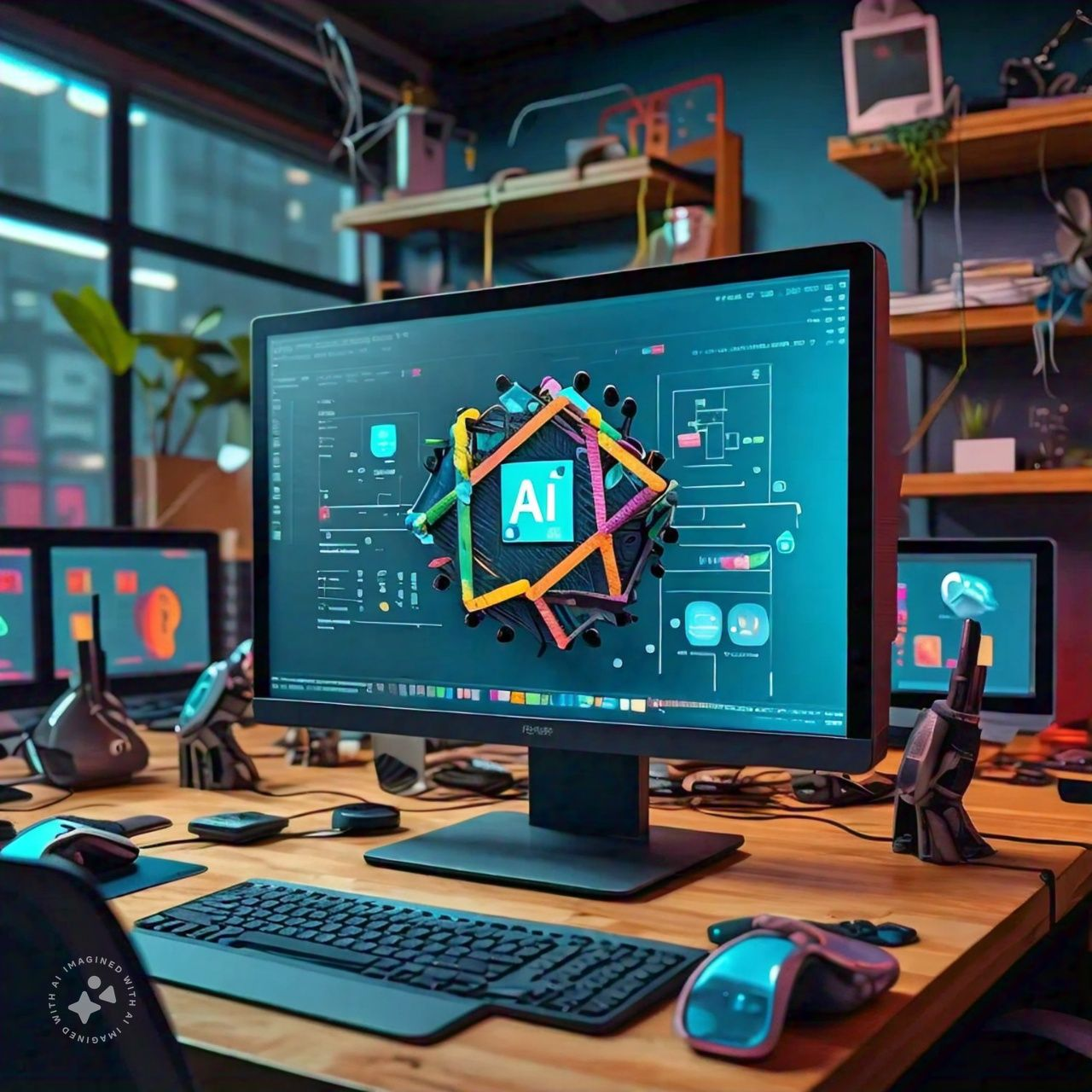
Bubble
Bubble is a standout no-code development platform that empowers users to create web apps with AI features. It seamlessly integrates with AI services, enabling the creation of complex workflows, task automation, and AI-powered user experiences without the need for coding.
Here are some other platforms that you can grab the information
- Helicone
- AIGur
- Blackbox
- Browse AI
- Brancher AI
- Levity
- Softr Studio
- InsightBase
- GeniusSheets (Myko AI)
- VFitterCreateML
- CreateML
- DataRobot
- Google AutoML
- RunwayML
- Google Teachable Machine
- Amazon SageMaker
- Microsoft Lobe
- Akkio
- Obviously AI
- Clarifai
- Polymer
Use Cases for No-Code AI
Marketing Automation
AI, through its machine learning algorithms, can automate repetitive tasks such as email marketing, ad targeting, and customer segmentation. For instance, tools like MonkeyLearn can analyze customer feedback or social media mentions, a process that would take hours for a human, to provide valuable insights for businesses.
Customer Support
No-code AI platforms empower customer support teams to create chatbots that handle customer queries, improve response times, and provide 24/7 customer support. This reduces the need for human intervention and enhances customer satisfaction, making the team more efficient.
Sales Prediction
AI models can analyze historical sales data to predict future trends. No-code platforms like Google AutoML allow businesses to use AI for better decision-making without the need for a data science team.
Content Creation
AI tools save time for content creators by helping them generate ideas, automate image tagging, or even generate blog posts. This speeds up the creative process and allows creators to focus on what matters most, making them more efficient and focused.
The Future of No-Code AI
As no-code AI platforms continue to evolve, we can expect more features and improved accessibility in the types of AI solutions that can be built. This revolution in AI technology will empower individuals and businesses to innovate, solve problems, and make data-driven decisions without needing to write any code. AI ethics jobs are essential for ensuring that technology is developed responsibly and moderately. Moonpreneur’s initiatives emphasize the need for ethical leadership in AI, preparing future innovators for this important work.
Future Trends in No-Code AI
New Technologies:
1. AutoML Integration: Automated Machine Learning (AutoML) will make it easier to create and use AI models on no-code platforms.
2. NLP Improvements: Better Natural Language Processing (NLP) will help users build smarter applications, like chatbots.
3. Edge Computing: This will allow applications to process data closer to where it comes from, reducing delays.
Predictions:
1. Industry-Specific Solutions: No-code platforms will offer more solutions tailored to specific industries.
2. Adoption by SMEs: More small and medium businesses will use no-code AI to stay competitive as platforms become easier and cheaper.
3. Enhanced Explainability: Future platforms will focus on making AI decisions easier to understand, addressing transparency concerns.
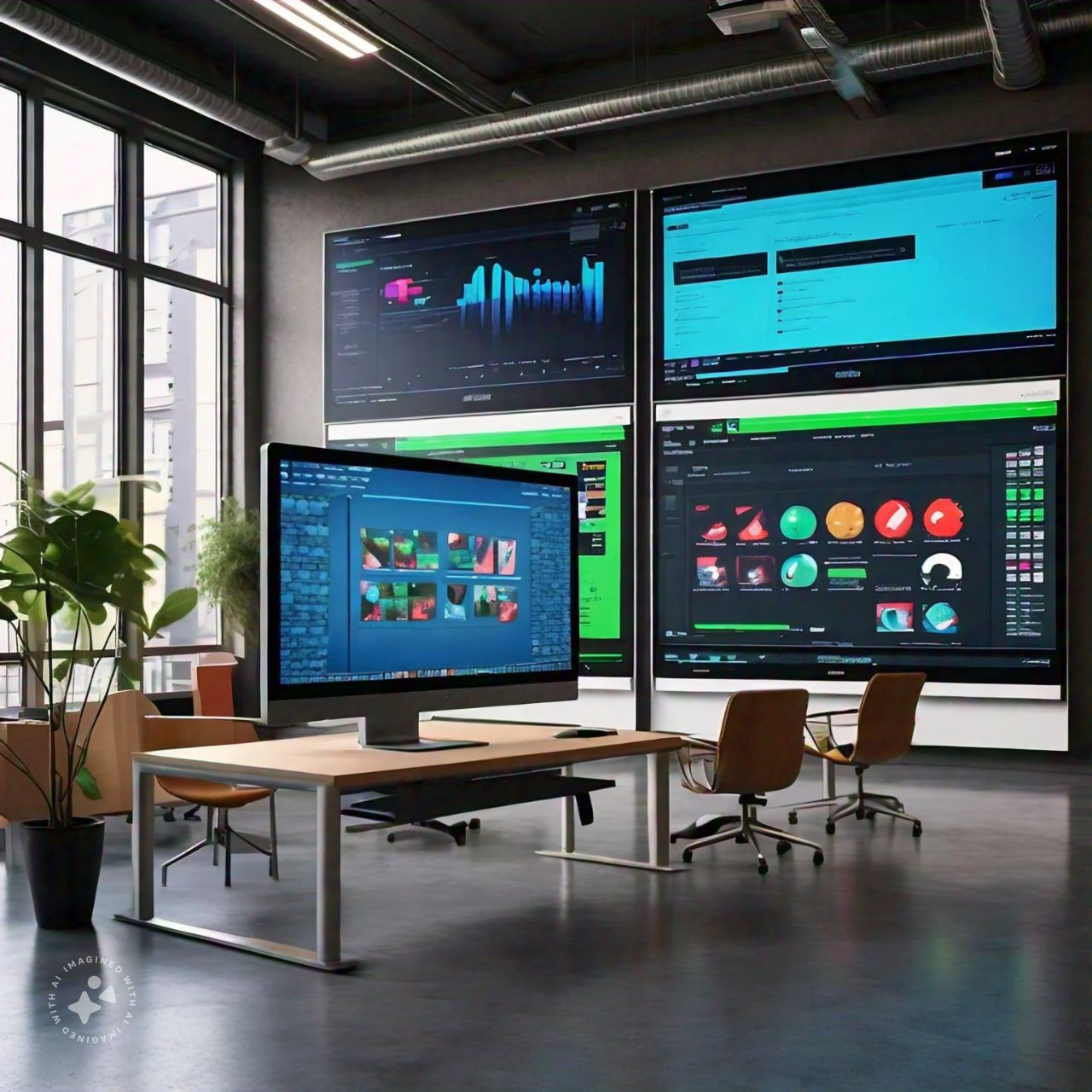
Challenges and Considerations
Challenges of No-Code AI | Tips for Success |
---|---|
1. Complex AI Models: Some AI models are complicated and need expert knowledge, making it hard for users. | 1. Training: Provide training for users to help them understand the no-code platform and AI basics. |
2. Security Concerns: No-code platforms should have strong security measures to prevent data breaches. | 2. Collaboration: Encourage teamwork between IT and business teams to ensure AI solutions meet company goals and technical standards. |
3. Customization Limits: Some platforms need to allow more customization, which can be an issue for organizations with specific needs. | 3. Updates: Regularly update the platform and AI models to keep them running well and secure. |
Advantages and Limitations of No-Code AI Platforms
Advantages of No-Code AI Platforms | Limitations of No-Code AI Platforms |
---|---|
No-code AI tools make advanced technology accessible. | No-code platforms struggle with highly complex AI tasks needing expertise. |
It reduces AI project costs, benefiting startups and businesses. | Data handling brings security risks, so ensure the platform follows laws. |
User-friendly platforms enable quick AI deployment, adapting to market changes. | Relying on platforms ties businesses to providers for updates, security. |
Better collaboration between teams. | Some platforms may struggle with scalability when handling large datasets. |
Encouragement for innovation and experimentation. | Pre-built models may offer generic solutions, lacking customization for specific needs. |
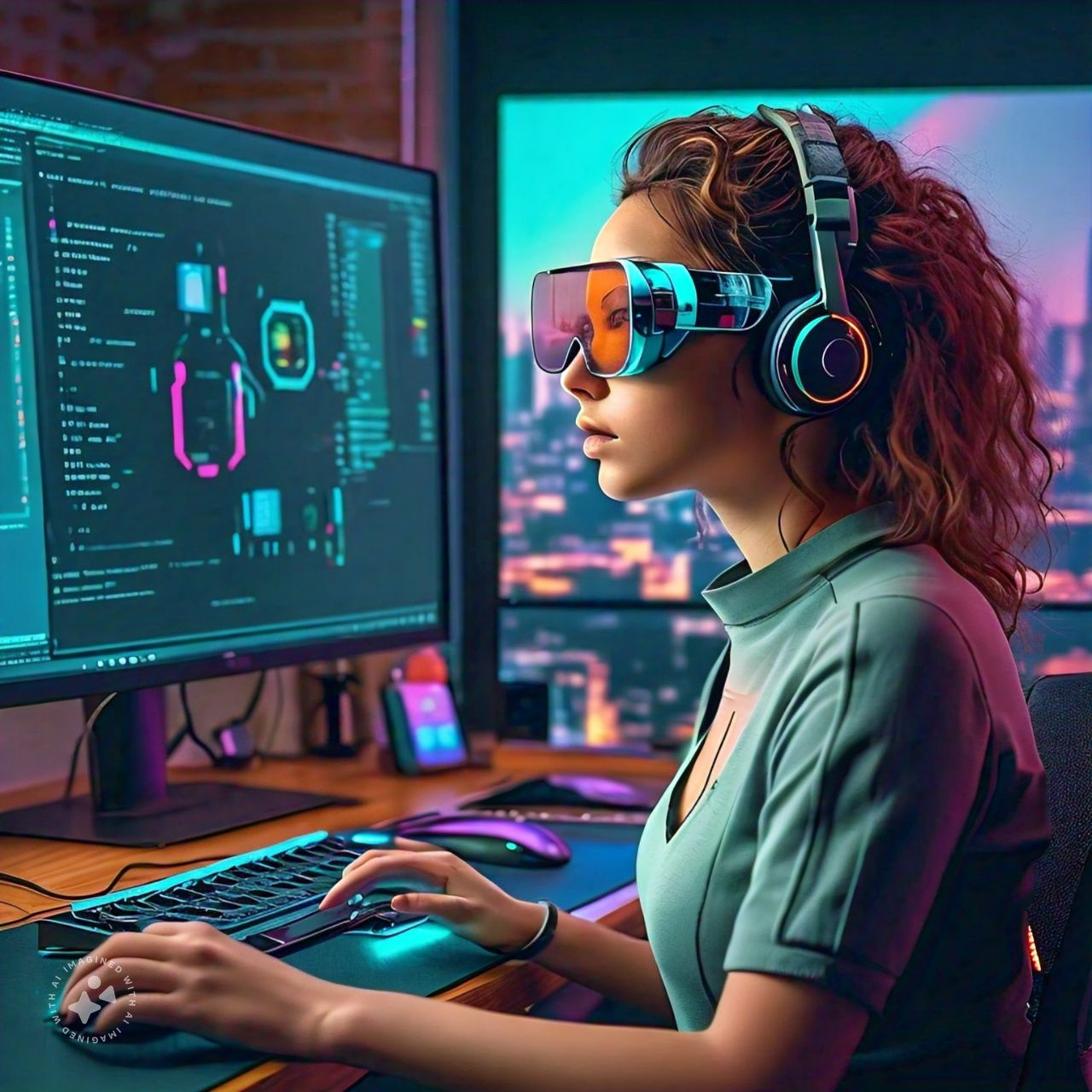
How to Choose the Right AI No-Code Platform
Choosing the right AI no-code platform for your business or project involves several key considerations. Here’s a structured approach to help you make an informed decision:
1. Identify Your Specific Needs and Goals
Objective Assessment. Determine what you want to achieve with AI. Are you looking to automate tasks, gain insights from data, enhance customer experience, or something else?
Use Case Identification. Identify specific use cases. Different platforms may excel in different areas like data analytics, process automation, chatbots, or predictive modeling.
2. Evaluate the Platform’s Capabilities
Assess the range of features offered. Does the platform support the types of AI models you need? Look for functionalities like data processing, machine learning algorithms, natural language processing, etc.
Check how much customization the platform allows. Can you tweak existing models or workflows to suit your unique requirements?
3. Consider Data Handling and Security
Look for platforms that easily integrate with your existing data sources and formats.
Ensure the platform adheres to data security standards and complies with relevant regulations (like GDPR, HIPAA, etc.), especially if you deal with sensitive or personal data.
4. Scalability and Performance
Choose a platform that can scale with your business needs. It should handle increasing amounts of data and more complex models over time.
Assess the performance capabilities of the platform, especially if you require real-time data processing or large-scale data analysis.
5. Support and Community
Good customer support is crucial. Check if the platform offers reliable technical support, training resources, and a knowledge base. A strong user community can be an invaluable resource. Look for platforms with an active user community, forums, and collaborative opportunities.
6. Cost Considerations
Understand the pricing model of the platform. Is it subscription-based, pay-as-you-go, or does it have a free tier? Make sure the costs align with your budget and the expected ROI. Be aware of any additional costs, such as extra features, increased data usage, or additional user licenses.
7. Trial and Evaluation
Before committing, use free trials or demos to test the platform. This hands-on experience can help you gauge whether the platform meets your needs and is a good fit for your team.
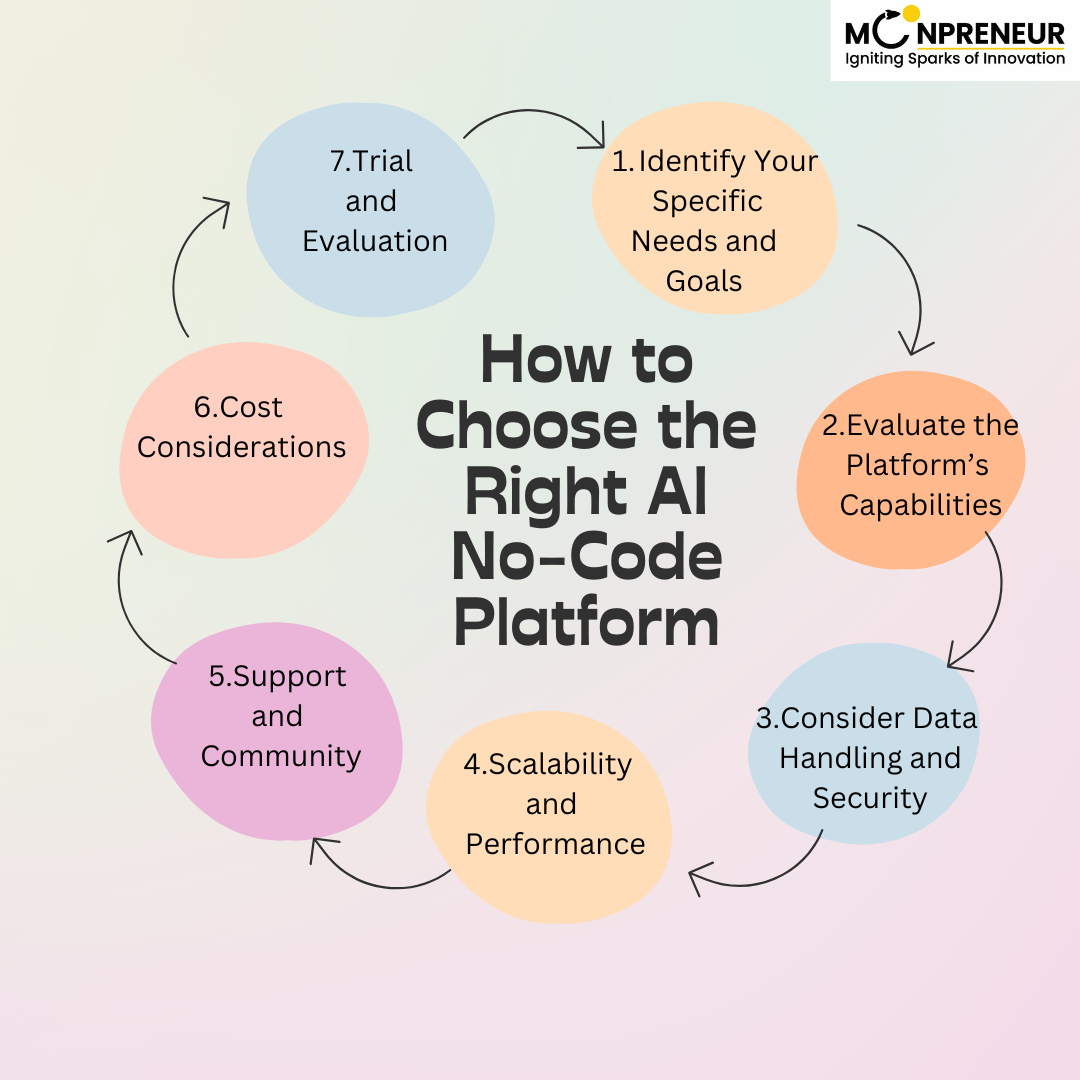
Case Study
Simplifying Customer Experience: Erie Insurance
Platform: Mendix
Implementation: Erie Insurance faced a challenge because they didn’t have many mobile developers. They decided to use Mendix, a low-code platform, to create a mobile app for their customers. This app allowed users to log in using biometrics (like a fingerprint), access their policy information, and view digital ID cards easily.
Impact:
- Cost Savings: By using Mendix, Erie Insurance saved money because they didn’t need specialized mobile developers.
- Faster Development: The app was developed quickly, allowing Erie Insurance to launch it to customers sooner.
- Improved User Experience: The app provided a smooth experience for users, making them happier and more engaged with the company.
Case Study Link: Mendix Case Study
Here are other case studies where you can go through it.
- Rapid Prototyping for Startups
- Streamlined Operations for NC State University
Final Thoughts
No-code AI represents a significant leap forward in making artificial intelligence accessible to everyone. By removing the coding barrier, businesses can adopt AI faster and more affordably, unlocking new opportunities for growth and innovation. Whether you’re looking to improve efficiency, enhance customer experiences, or drive business growth, no-code AI platforms provide the tools to bring your ideas to life effortlessly.
Role of AI in Augmented and virtual reality by improving user experiences and making interactions more intuitive. It helps create realistic environments and adaptive content that respond to user actions.
Moonpreneur is on a mission to disrupt traditional education and future-proof the next generation with holistic learning solutions. Its Innovator Program is building tomorrow’s workforce by training students in AI/ML, Robotics, Coding, IoT, and Apps, enabling entrepreneurship through experiential learning.