The rapid advancement of technology has transformed various industries, making machine learning (ML) a pivotal field today. As we approach 2025, the demand for machine learning careers is not just growing, but expected to skyrocket. This blog delves into the emerging roles in machine learning, the essential skills required, and how to prepare for a successful career in this dynamic sector.
The Growing Importance of Machine Learning
According to the U.S. Bureau of Labor Statistics, jobs in the field of machine learning and artificial intelligence are projected to grow by 22% from 2020 to 2030, much faster than the average for all occupations.
McKinsey & Company report noted that over 70% of companies surveyed were actively adopting AI technologies, highlighting the urgency for professionals skilled in machine learning.
Key Roles in Machine Learning Careers
As the field of machine learning continues to evolve, it’s giving rise to a variety of new and exciting roles. Here, we’ll take a closer look at some of the key positions that are set to make a significant impact in 2025:
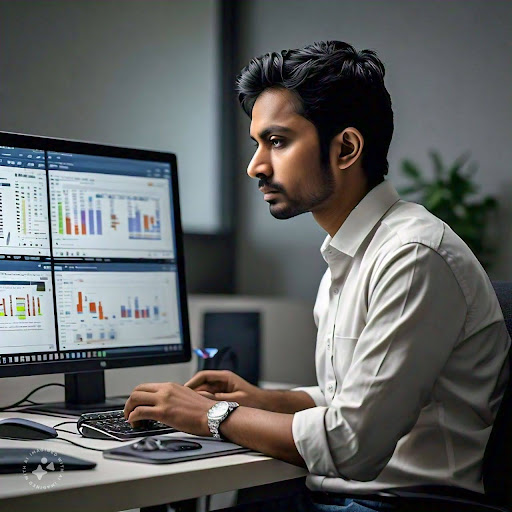
Data scientists are at the forefront of an exciting field, extracting insights from large datasets. The Harvard Business Review has even dubbed data science the “sexiest job of the 21st century.”
Responsibilities:
- Analyze and interpret complex data sets to inform business decisions.
- Build predictive models and algorithms.
- Collaborate with cross-functional teams to understand data requirements.
Career Path: Data scientists have a world of opportunities ahead. They can progress to roles like Senior Data Scientist, Data Science Manager, or even Chief Data Officer.
Machine learning engineers play a crucial role in the industry, focusing on implementing algorithms and building scalable models. They are the ones who bridge the gap between data science and software engineering, making them an integral part of the field. A report from LinkedIn highlighted that the demand for machine learning engineers increased by 32% between 2019 and 2021, further underlining their importance.
Responsibilities:
- Design, implement, and optimize machine learning models.
- Deploy models into production and monitor their performance.
- Work closely with data scientists to refine models and algorithms.
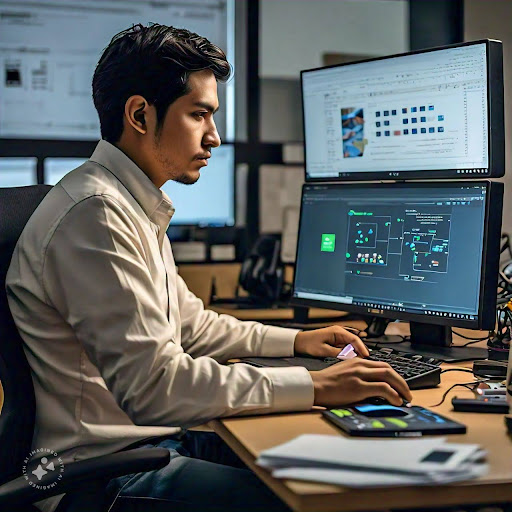
Recommended Reading : What are the Future Machine Learning Jobs?
Career Path: The field of machine learning offers a promising career path. As a machine learning engineer, you can advance to positions like Senior Machine Learning Engineer, AI Architect, or Director of AI, providing you with ample opportunities for growth and development.
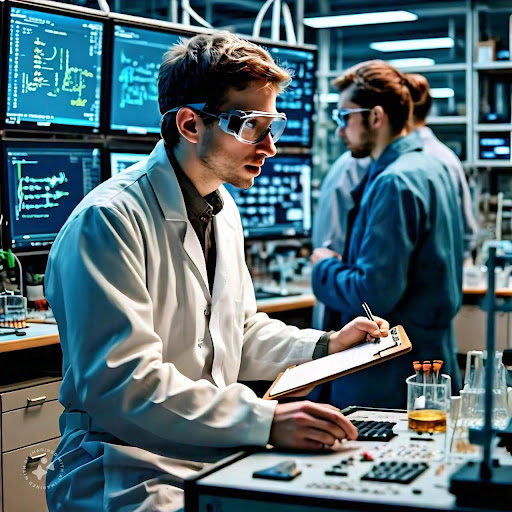
Recommended Reading : AI Strategist: Guiding Businesses Through the AI Revolution
AI research scientists, the trailblazers of innovation, are constantly pushing the boundaries of what machine learning can achieve. Their work in academic or corporate research labs, such as the groundbreaking efforts of OpenAI in natural language processing, inspires and motivates the entire AI community.
Responsibilities:
- Conduct innovative research to develop new algorithms and approaches.
- Publish research findings in academic journals and conferences.
- Collaborate with other researchers and industry professionals on advanced projects.
Career Path: AI research scientists typically hold advanced degrees in computer science, mathematics, or a related field. They also need strong analytical and problem-solving skills, as well as a deep understanding of machine learning and data analysis. With these qualifications, they can move into roles such as Principal Research Scientist, Research Director, or AI Policy Advisor.
Business intelligence (BI) developers, by leveraging machine learning, play a crucial role in boosting productivity. According to Gartner, companies that invest in BI capabilities experience up to a 30% increase in productivity, underlining the significant impact of BI on business operations.
Responsibilities:
- Create and manage BI tools and dashboards for data visualization.
- Analyze business data to derive actionable insights.
- Collaborate with stakeholders to align BI initiatives with business goals.
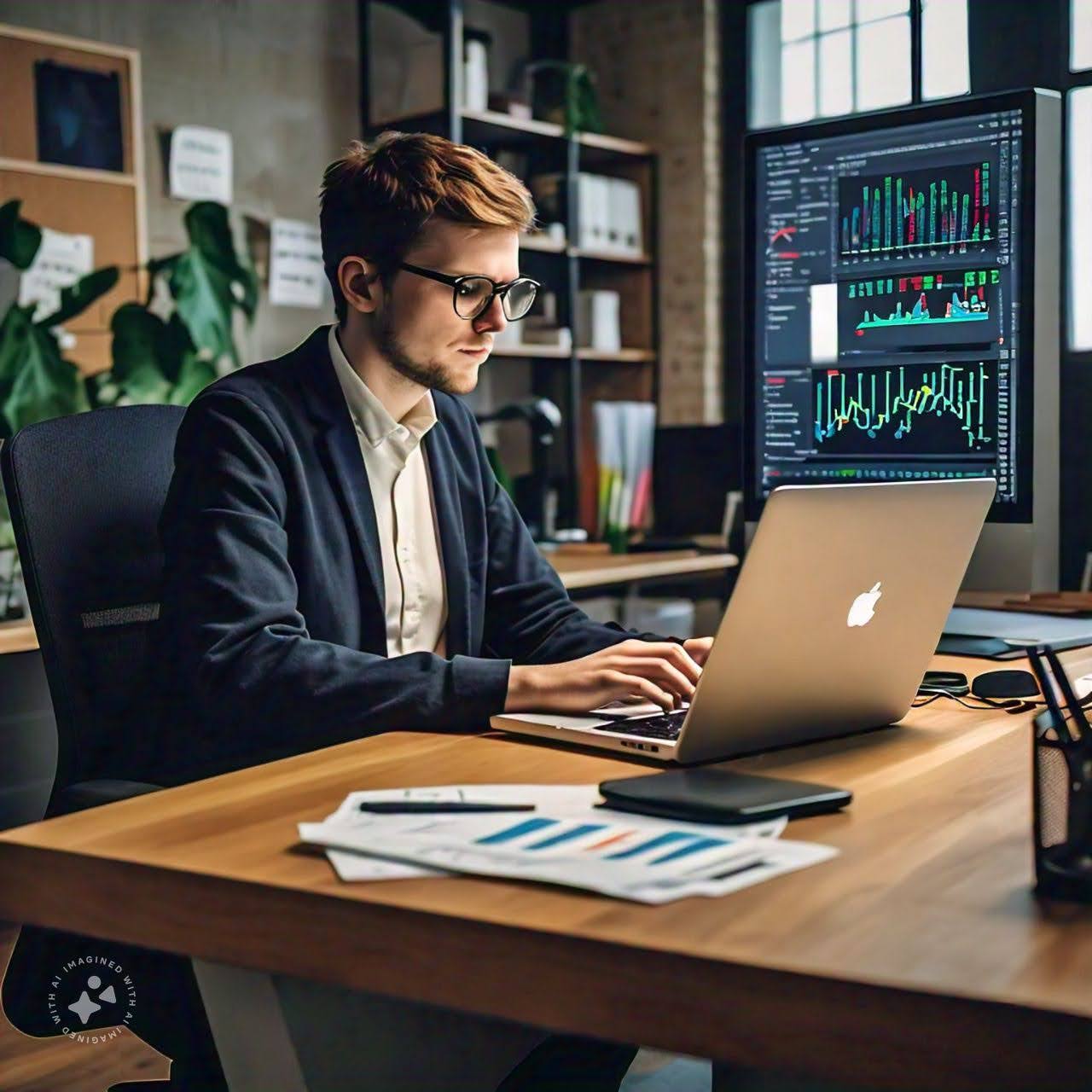
Career Path: The career path for business intelligence developers is promising. With the right skills and experience, they can transition to roles such as BI Manager, Data Analytics Consultant, or even Chief Data Officer.
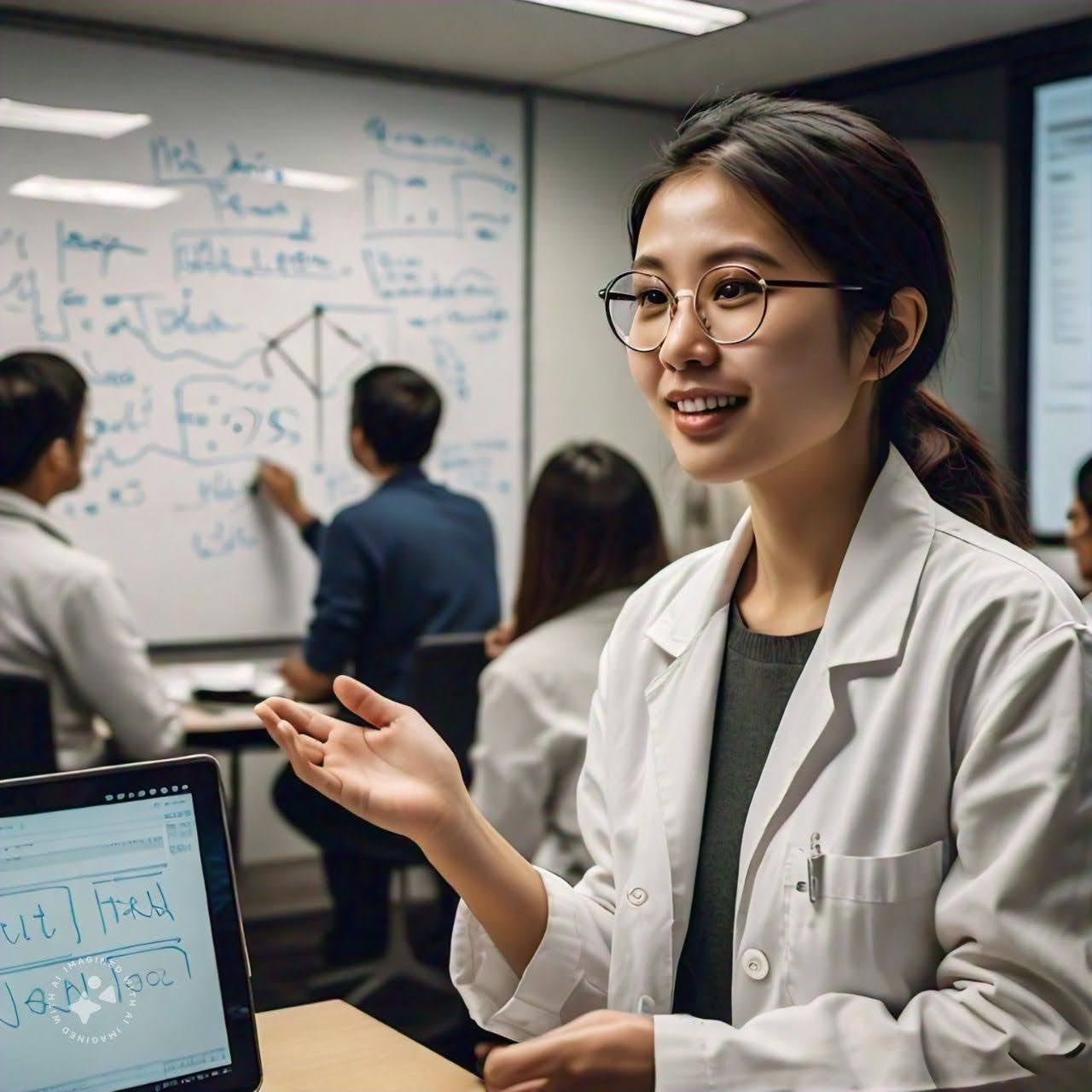
MLOps is an exciting and rapidly growing discipline that focuses on the deployment and maintenance of machine learning models. The 2021 State of ML Ops report by Algorithmia revealed that 60% of companies are prioritizing MLOps to scale their machine learning efforts, indicating a promising future for those considering a career in this field.
Responsibilities:
- Ensure the smooth deployment and operation of machine learning models in production.
- Monitor model performance and implement improvements.
- Collaborate with data scientists and IT teams to streamline workflows.
Career Growth: MLOps engineers have a clear path for advancement. They can progress to roles such as Senior MLOps Engineer, AI Operations Manager, or even Head of AI Infrastructure, offering a promising and rewarding career trajectory.
As AI continues to transform the way we live and work, a multitude of unique roles are emerging, from data scientists to AI ethicists. Whether you’re a tech lover or a creative thinker, there’s a role in this vibrant field that’s just right for you. Eager to uncover the thrilling career paths in machine learning? Read More to find your ideal role! |
Case Study: Google DeepMind
Google DeepMind, founded in 2010 and acquired by Google in 2014, is a leading AI research lab known for its advancements in machine learning, intense learning and reinforcement learning. Its mission is to “solve intelligence and then use that to solve everything else.”
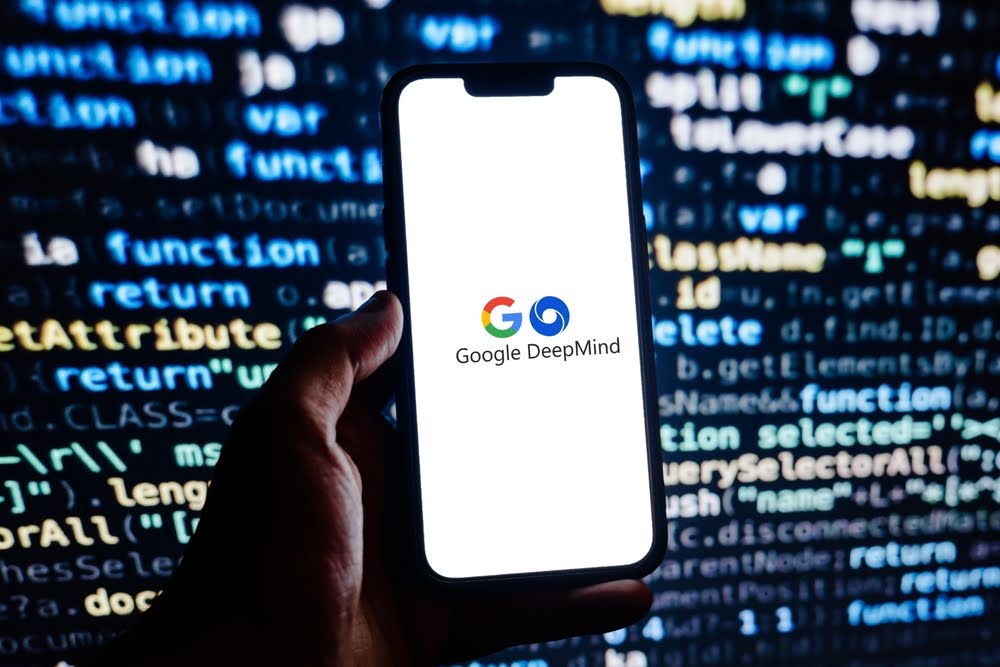
Key Achievement: AlphaGo
One of DeepMind’s landmark achievements is AlphaGo, an AI program designed to play the complex board game Go. In March 2016, AlphaGo’s victory over Lee Sedol, a top Go player, in a five-game match, resonated globally.
Skills Required for Machine Learning Careers
Technical Skills | Soft Skills |
---|---|
Programming Languages: Proficiency in languages such as Python, R, and Java is crucial for data manipulation and model development. | Critical Thinking and Problem-Solving: Ability to analyze complex problems and devise effective solutions is crucial in machine learning. |
Understanding Algorithms and Data Structures: A solid grasp of algorithms and data structures is necessary for optimizing ML models. | Communication Skills: Being able to explain complex concepts to non-technical stakeholders is essential for driving initiatives forward. |
Experience with ML Frameworks: Familiarity with frameworks like TensorFlow and PyTorch simplifies the model-building process. | Collaboration and Teamwork: Machine learning often involves interdisciplinary teamwork, making strong collaboration skills vital. |
As the field evolves, unique career paths are emerging, including:
1. Ethical AI Specialist
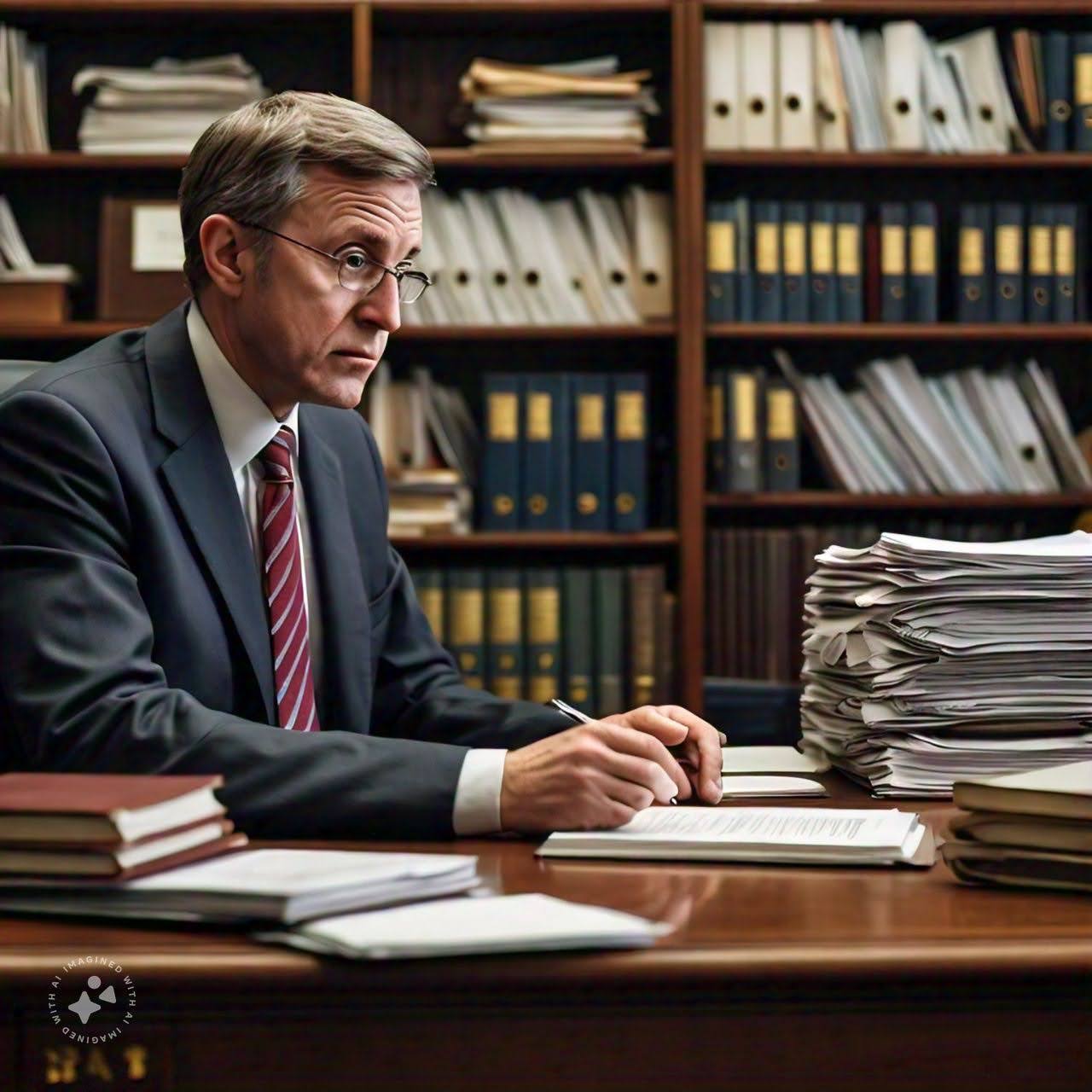
With the increasing concerns about bias and ethics in AI, ethical AI specialists are playing a crucial role in shaping the future. Their focus on ensuring that machine learning applications adhere to ethical standards, such as with IBM’s AI Fairness 360 toolkit, is not just a job, but a mission with a profound impact on society.
Responsibilities:
- Ensure that AI and machine learning applications adhere to ethical standards.
- Analyze algorithms for bias and fairness.
- Advocate for responsible AI practices within organizations.
Career Path: The field of ethical AI is not just about the present, but also about the future. Ethical AI specialists can progress to exciting and impactful roles like AI Policy Advisor or Director of Ethical AI, offering a hopeful and exciting future for those interested in this field.
2. Data Privacy Analyst
Data privacy analysts are entrusted with the vital task of protecting user data in machine learning applications. Their work is crucial, as a Deloitte survey found that 88% of consumers are concerned about data privacy. The role of data privacy analysts is not just about compliance, but also about building and maintaining user trust, which in turn, impacts business reputation.
Responsibilities:
- Ensure compliance with data protection regulations, such as GDPR and CCPA.
- Develop and implement data privacy policies and practices.
- Conduct risk assessments and audits to identify potential data vulnerabilities.
- Educate stakeholders on data privacy best practices.
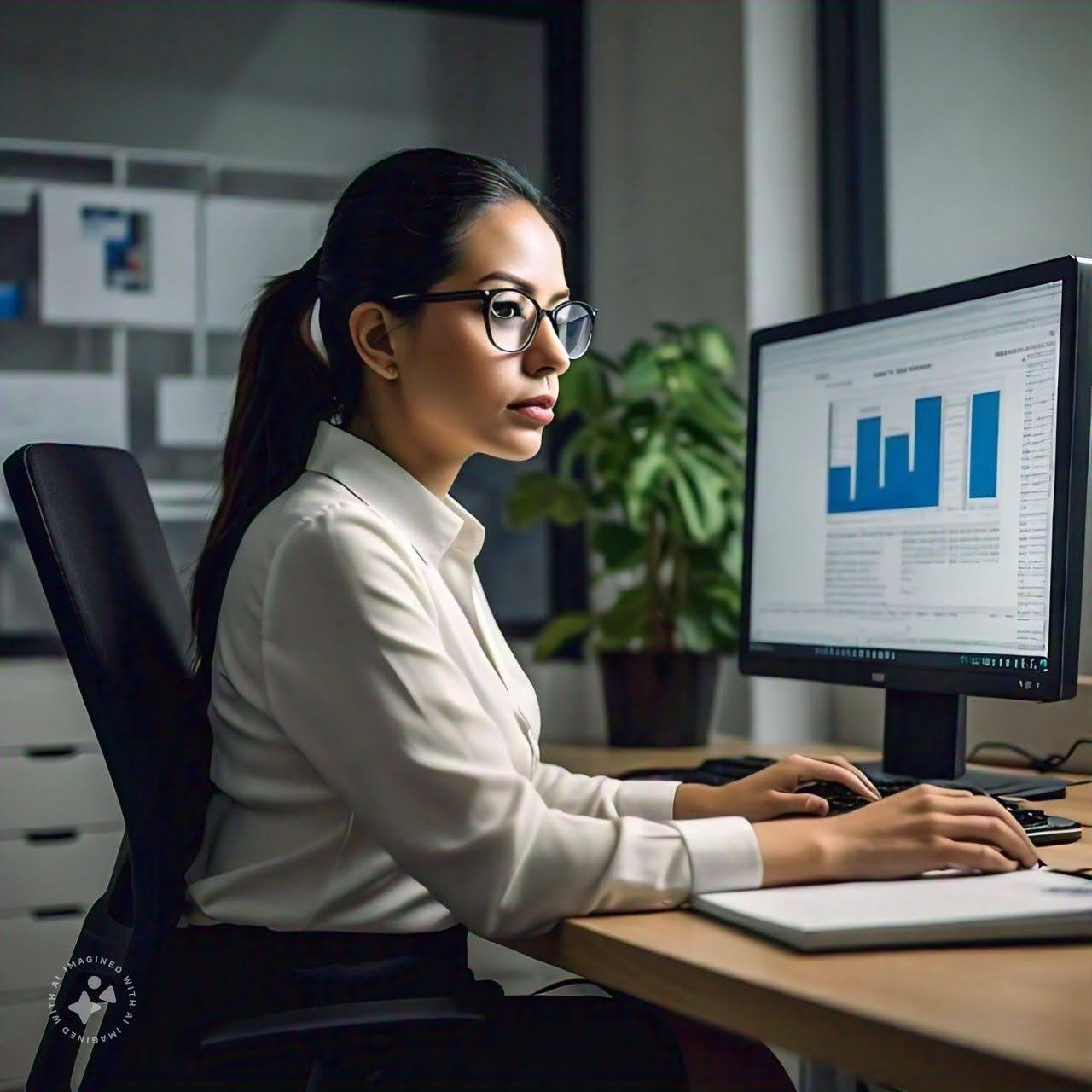
Recommended Reading : Data Science For Kids: Tips and Techniques
Career Path: Data privacy analysts have a promising career trajectory. With dedication and expertise, they can advance to roles like Data Privacy Manager, Chief Privacy Officer, or Compliance Director, offering a path of growth and development.
3. AI Policy Advisor
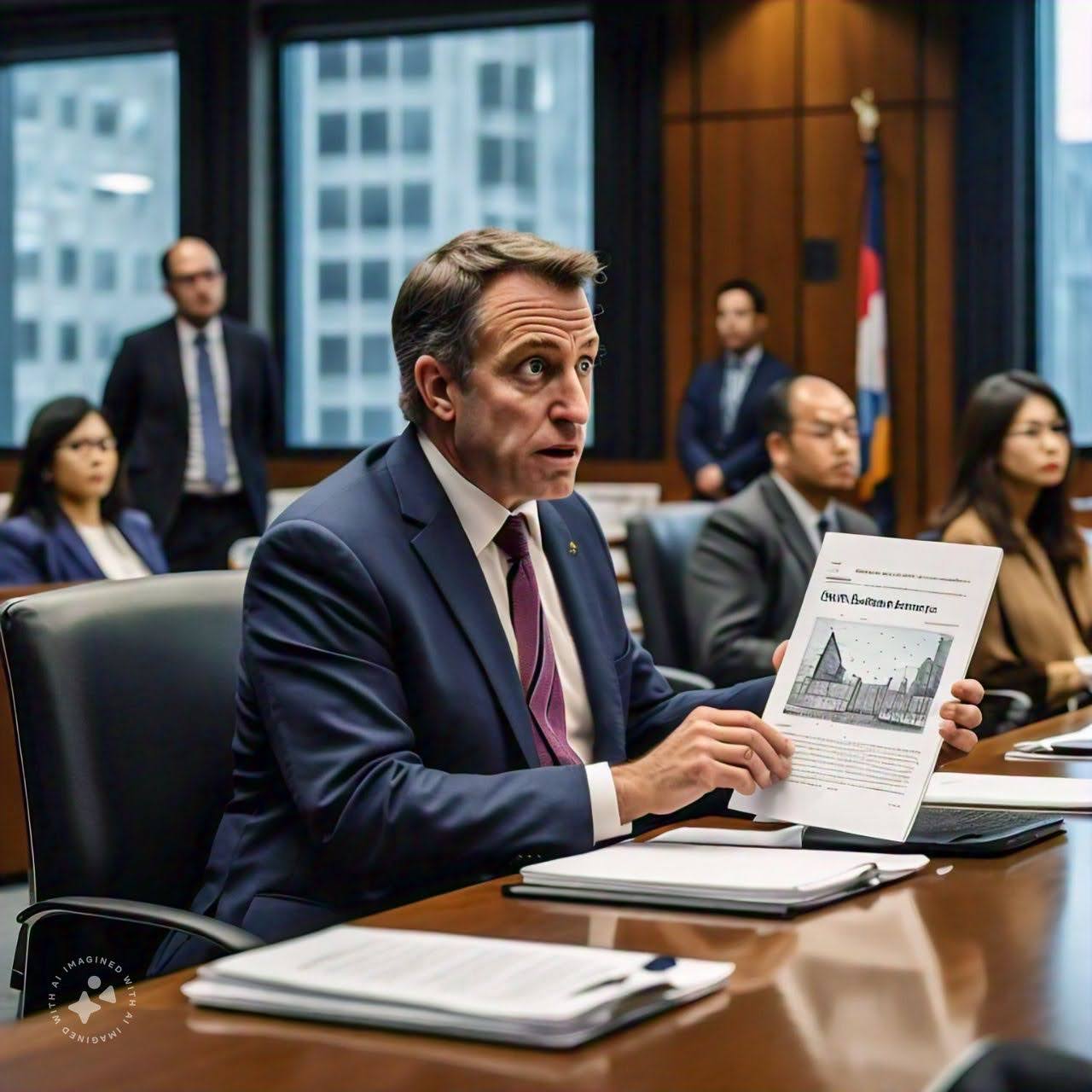
According to a 2021 report by the World Economic Forum, over 65% of job postings in AI and ML require at least a bachelor’s degree. |
AI policy advisors hold a pivotal role in shaping regulations and policies related to AI and machine learning technologies. Their work is of utmost importance as they collaborate with government agencies and organizations to create frameworks that govern the responsible use of AI, thereby significantly impacting the future of these technologies.
Responsibilities:
- Analyze the impact of AI technologies on society and recommend policies.
- Collaborate with stakeholders to develop ethical AI guidelines.
- Advocate for responsible AI practices and regulations.
- Monitor legislative developments and advise organizations on compliance.
Career Path: AI policy advisors have a promising career path. They can progress to positions such as Senior Policy Analyst, Director of AI Policy, or Chief Regulatory Officer, offering a bright future in the dynamic field of AI policy.
Future Trends Shaping Machine Learning Careers
Looking ahead, several trends are set to influence machine learning careers:
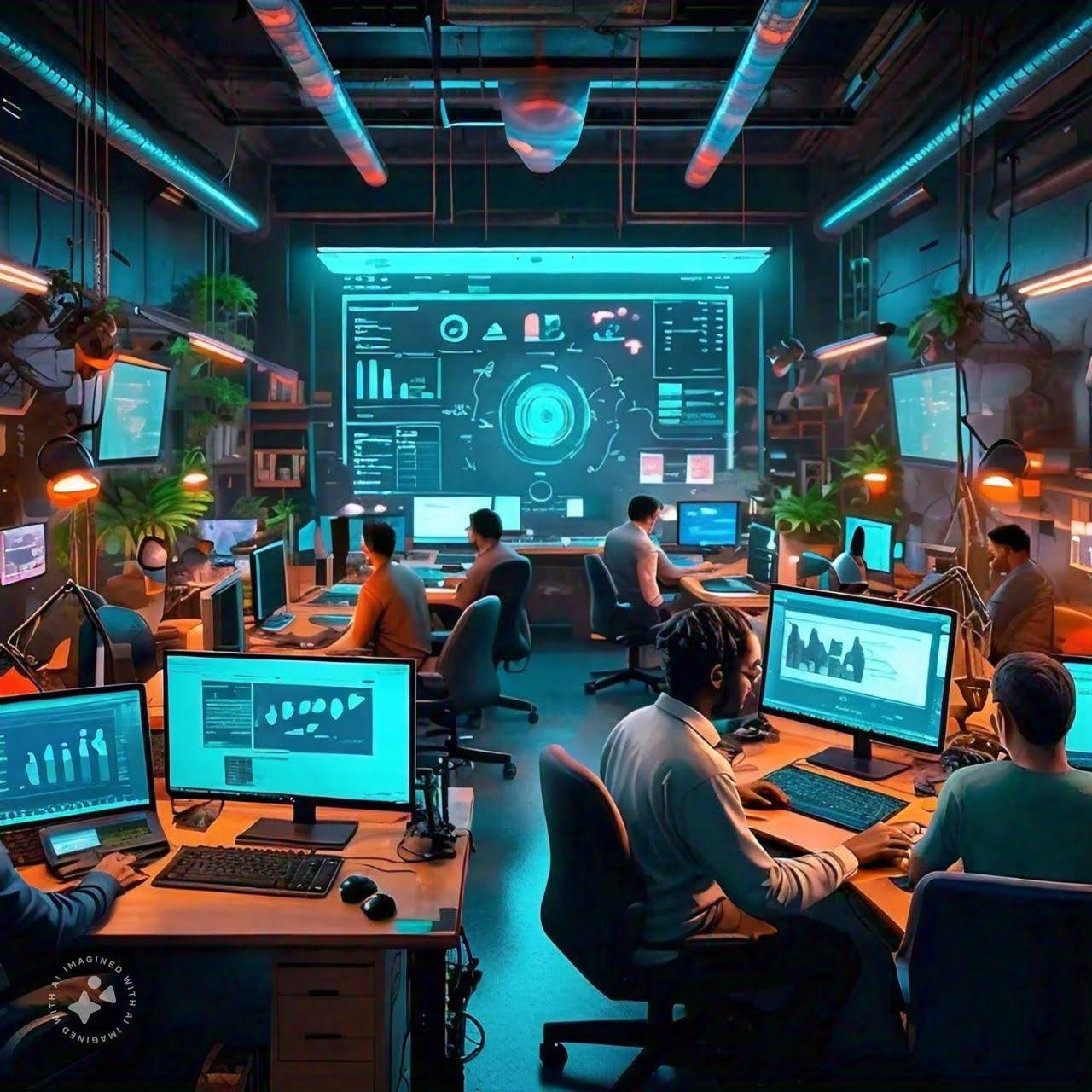
- Integration of ML in IoT Devices: The Internet of Things (IoT) is a key driver in the increasing demand for machine learning solutions. This technology, which is expected to connect 30 billion devices by 2030, according to Statista, is creating a multitude of job opportunities in the field of machine learning.
- Growth of Autonomous Systems: As autonomous systems become more prevalent, the role of professionals in developing and maintaining these systems becomes increasingly crucial. Tesla’s advancements in self-driving technology are a testament to the impact of these professionals on the industry.
- Predictions for Job Roles: As Technology Advances, we can expect new job roles to emerge by 2030. This shift will necessitate continuous learning and adaptability. The World Economic Forum predicts that while 85 million jobs may be displaced by a shift in labor between humans and machines, 97 million new roles could emerge, underscoring the importance of these skills in the future job market.
How to Prepare for a Career in Machine Learning
Getting ready for a career in machine learning can be an exciting journey! It’s a field that’s always changing, and there are plenty of opportunities to dive in. Here are some practical steps you can take to set yourself up for success:
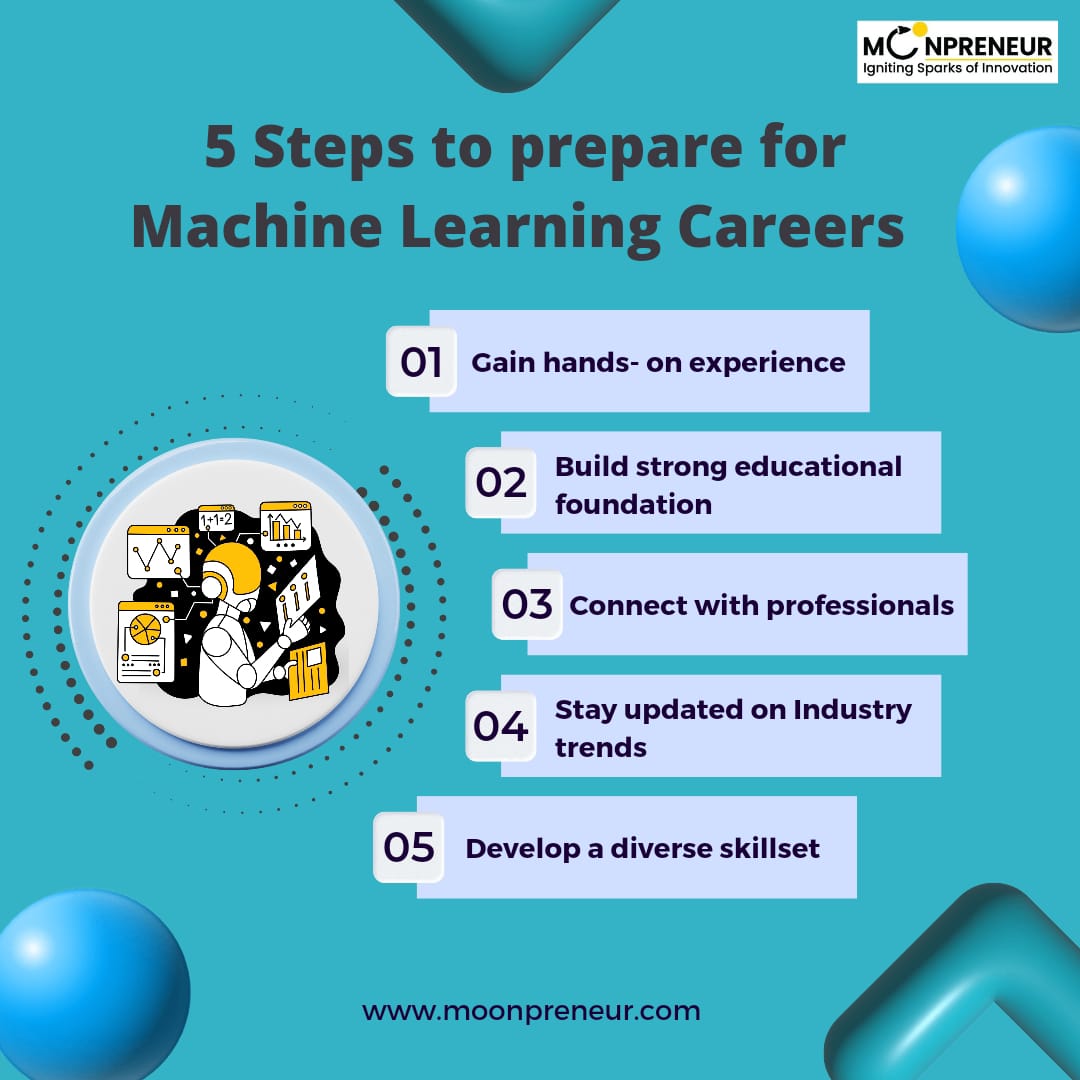
1. Gain Hands-on Experience
One of the best ways to learn is by doing. Here’s how you can get some hands-on experience:
- Join Online Competitions: Websites like Kaggle offer fun competitions where you can tackle real-world problems and see how you stack up against others. It’s a great way to learn and build your portfolio!
- Work on Personal Projects: Try starting your machine learning projects. Pick a dataset you’re interested in—maybe something related to sports stats or social media trends—and build a model around it. Websites like the UCI Machine Learning Repository have tons of datasets to explore.
- Look for Internships: Don’t underestimate the power of internships! They can give you practical experience and help you understand how machine learning works in a professional setting.
2. Build a Strong Educational Foundation
While hands-on experience is key, having a solid educational background will definitely give you an edge:
- Consider Relevant Degrees: A degree in computer science, data science, or statistics can provide you with the foundational knowledge you need in algorithms and programming.
- Take Online Courses: Fantastic courses are available on platforms like Coursera or edX. Andrew Ng’s Machine Learning course is a classic and covers the essentials.
3. Network and Connect with Professionals
Building a network is super important in any field, and machine learning is no exception:
- Attend Meetups and Conferences: Look for local meetups or attend conferences like NeurIPS or ICML. These events are great for meeting people and learning about the latest in the industry.
- Engage on LinkedIn: Create a LinkedIn profile and connect with people working in machine learning. Follow industry leaders and get involved in discussions to keep your knowledge up to date.
4. Stay Updated on Industry Trends
Machine learning is constantly evolving, so it’s crucial to keep your finger on the pulse:
- Follow Blogs and Podcasts: Subscribe to blogs like Towards Data Science or podcasts like The AI Alignment Podcast to stay informed about the latest trends and advancements.
- Read Research Papers: If you want to dive deeper, check out research papers on sites like arXiv or Google Scholar. They can provide valuable insights into cutting-edge developments in machine learning.
5. Develop a Diverse Skill Set
Alongside technical skills, soft skills are just as critical:
- Master the Technical Skills: Focus on learning programming languages like Python and R and get comfortable with machine learning frameworks such as TensorFlow or PyTorch.
- Hone Your Soft Skills: Skills like critical thinking, problem-solving, and communication will serve you well, especially when you need to work in teams or present your findings.
Conclusion
In conclusion, pursuing a career in machine learning offers numerous opportunities in a rapidly growing field. With the right skills, education, and preparation, you can carve out a successful career in this dynamic landscape. As illustrated by the success of Google DeepMind, machine learning professionals are shaping the future of technology and creating solutions to complex challenges. Embrace the journey, stay curious, and prepare for the exciting career ahead!
Moonpreneur is on a mission to disrupt traditional education and future-proof the next generation with holistic learning solutions. Its Innovator Program is building tomorrow’s workforce by training students in AI/ML, Robotics, Coding, IoT, and Apps, enabling entrepreneurship through experiential learning.
The blog gives a great overview of potential career paths in machine learning, but I think it would be interesting to dive deeper into the practical aspects of transitioning into the field. For example, how can someone with a non-technical background, like a humanities major, pivot into machine learning? What are some entry-level projects or skills that could make this transition smoother? It would be helpful to explore how people are building their portfolios from scratch while learning.
Thanks for the insightful comment! To address your point, transitioning into machine learning from a non-technical background is definitely possible. Many people begin by focusing on foundational skills like statistics, programming (especially Python), and machine learning basics. Starting with small projects, such as data analysis or building simple models using datasets from platforms like Kaggle, can be a great way to build experience. Gradually, they can move on to more complex challenges as they grow in confidence. It’s all about taking those first steps and learning continuously.
It’s great to see an emphasis on the variety of career opportunities in machine learning. One thing I wonder, though, is how the increasing use of AI and automation will impact job opportunities within the field. While machine learning engineers are in high demand now, could this shift as AI systems become more self-sufficient? It’d be interesting to consider how machine learning professionals can future-proof their careers by adapting to these changes.
That’s a great observation! As AI continues to advance, some aspects of machine learning may indeed become more automated. However, this shift emphasizes the need for machine learning professionals to specialize in areas such as AI ethics, model interpretability, and custom model development. As the field evolves, there will always be a demand for professionals who can understand the broader implications of AI and ensure these technologies are applied responsibly and innovatively. It’s a great idea to stay adaptable and continuously upskill in emerging areas.