The healthcare industry is undergoing a significant transformation driven by the rapid advancement of Artificial Intelligence (AI). Generative AI, as a subset of AI, has emerged as a game-changer in healthcare, enabling machines to generate new and original content, such as images, text, or predictions. This technology has the potential to revolutionize patient care, and importantly, it can significantly improve health outcomes, providing reassurance about the quality of healthcare. In this blog, you will read about the different aspects of Generative AI and how the implementation of Generative AI in Healthcare can bridge the gap between data and diagnosis.
What is Generative AI?
Imagine you’re an artist with a magical paintbrush. Instead of manually creating every stroke on a canvas, you tell the brush your vision—whether it’s a beautiful sunset or a futuristic city. The brush then starts creating it for you, filling in the details, adding textures, and even surprising you with ideas you hadn’t thought of. Generative AI is like that magical paintbrush, but it works for everything, including text, music, art, code, and beyond. It’s not limited to a single field, it’s adaptable and can be applied in various industries. It uses complex algorithms, such as Generative Adversarial Networks (GANs) and Variational Autoencoders (VAEs), to learn patterns in data and generate new insights. Let’s explore its uses in Healthcare.
Benefits of Generative AI in Healthcare
Generative AI is revolutionizing healthcare by leveraging its ability to analyze data, create new insights, and personalize patient care. Its unique capabilities are helping healthcare providers address challenges more efficiently and offer better outcomes for patients. Here are a few key benefits of using generative AI in healthcare:
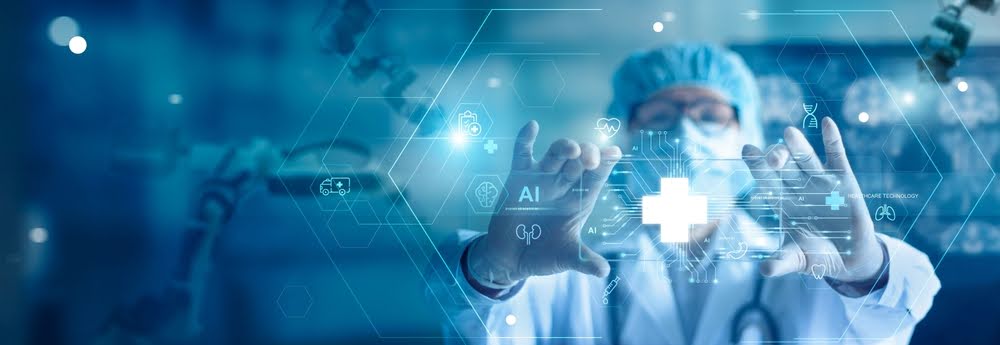
1. Analyze large datasets to identify trends and patterns: Generative AI analyzes medical data (such as patient records, clinical trials, and research studies) faster and more accurately than humans. By analyzing these datasets, it identifies critical trends and patterns that might be difficult to spot manually. For example, it can detect early signs of disease outbreaks, predict treatment outcomes, or find correlations between lifestyle factors and health conditions.
2. Generate synthetic data to supplement limited datasets: Another key benefit of generative AI in healthcare is its ability to generate synthetic data. In healthcare, there is often a scarcity of sufficient data, especially for rare diseases or new treatments. Generative AI can create synthetic data that mimics accurate patient data while maintaining privacy. This synthetic data can be used to train machine learning models, run simulations, and test hypotheses without needing large volumes of actual patient data.
3. Develop personalized models for patient care: Generative AI is not just about predicting treatments, it’s about shaping a future where patients receive the right treatment at the right time, potentially reducing side effects and improving recovery times. By analyzing an individual’s unique health data such as genetics, medical history, and lifestyle factors, it predicts what treatments or preventive measures would be most effective for them. This personalized approach ensures that patients receive the right treatment at the right time, potentially reducing side effects and improving recovery times.
Real-World Applications in Healthcare
Generative AI is making transformative strides in healthcare, improving everything from diagnosis to personalized treatments. Here’s a closer look at three key areas where generative AI is making a significant impact:
1. Medical Imaging: Enhancing Accuracy and Speed
Medical imaging is a field where generative AI is not just showing potential, but also instilling hope for the future of healthcare. Traditionally, interpreting complex medical images like MRIs, X-rays, or CT scans requires specialized expertise, and even then, abnormalities can be missed due to human error or fatigue. Generative AI, however, is enhancing image quality, detecting abnormalities, and assisting in diagnosis, paving the way for a more accurate and efficient healthcare system.
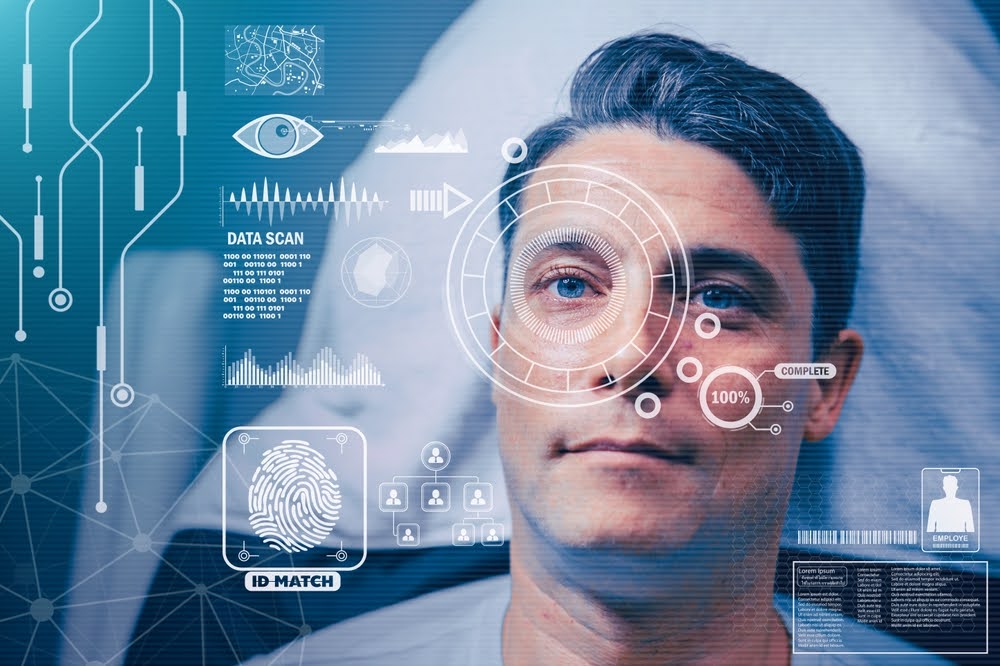
Example: Google’s AI-powered retinal scan analysis
This technology is a beacon of hope in the fight against diseases that can lead to blindness. By analyzing retinal images, the AI can detect early signs of diabetic retinopathy, often before symptoms manifest. This early detection capability not only reassures patients but also allows for timely intervention, significantly improving patient outcomes.
2. Personalized Medicine: A Shift to Individualized Treatment
Generative AI is revolutionizing personalized medicine by predicting how a patient might respond to a specific treatment. This prediction, based on the individual’s genetic makeup, lifestyle, and medical history, allows doctors to select the most effective therapies while minimizing side effects.
Example: At Stanford University, researchers are using AI to diagnose and treat cancer with greater precision. By analyzing genetic and molecular data from patients, AI systems can predict how a particular cancer will respond to various treatments. AI’s success in identifying which patients are likely to benefit from immunotherapy or targeted drug treatments instills confidence in its capabilities, leading to more successful outcomes in complex cases like cancer.
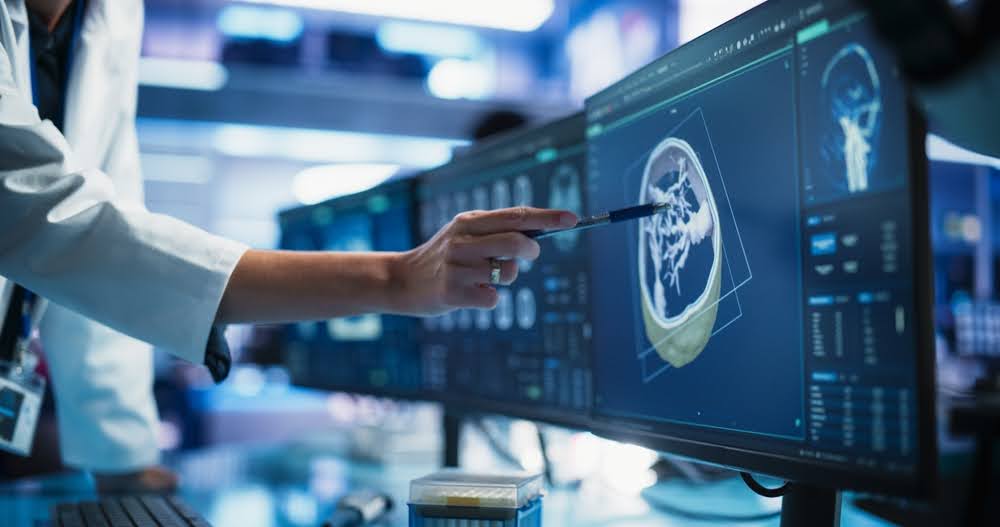
3. Clinical Decision Support: Empowering Physicians with Data-Driven Insights
AI-driven clinical decision support systems are transforming how healthcare providers diagnose and treat patients. These systems, known for their reliability, analyze large volumes of data, from patient records to clinical guidelines, and provide physicians with real-time recommendations. This not only speeds up the decision-making process but also ensures that medical professionals have access to the latest evidence-based information, fostering trust in the technology.
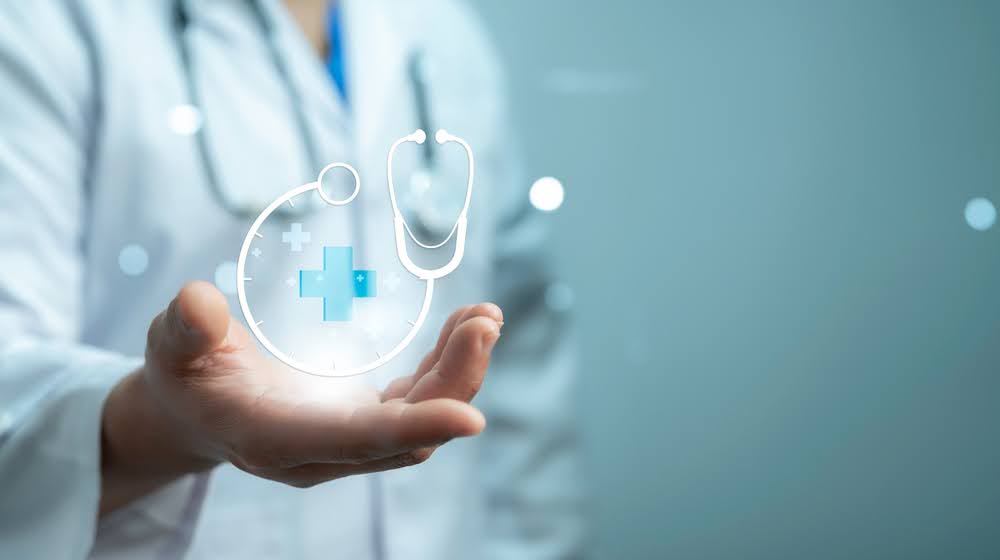
Example: The Mayo Clinic has been a pioneer in harnessing the power of AI for clinical decision support. Their system, which analyzes patient data, medical histories, and symptoms, is designed to suggest potential diagnosis or treatment paths. This empowers physicians to make more informed decisions, thereby reducing the risk of misdiagnosis and ultimately enhancing patient care.
Addressing Ethical Concerns and Bias Mitigation
When it comes to using AI in healthcare, there are significant challenges that need to be addressed for it to be fully effective and widely trusted. These challenges revolve around the quality of data, the transparency of AI systems, and the evolving regulatory frameworks that guide their use. Let’s take a closer look at these critical areas and the solutions being developed to address them.
Quality and Bias: Ensuring Diverse, Representative Data Sets
AI systems in healthcare are fundamentally reliant on the quality of the data they are trained on. The significance of this cannot be overstated, as it underscores the necessity for the data used to train AI models to be diverse and representative of different populations. This inclusivity is crucial to ensure that AI-driven solutions are effective for everyone. If the data is biased, it could lead to inaccurate predictions, particularly for those who aren’t well represented in the training data. For instance, an AI system primarily trained on data from Caucasian men might not perform as well when diagnosing diseases in women or people from other ethnic backgrounds.
Solution: Addressing the issue of data quality and bias in AI systems is a collaborative effort between healthcare providers and AI developers. This joint focus on data curation and validation processes is key to ensuring the accuracy and inclusivity of AI-driven solutions. It involves gathering a wide range of data from different populations, ensuring its cleanliness and accuracy, and then validating the AI models against real-world outcomes.
Transparency and Explainability: Understanding AI-driven Decisions
One of the most pressing concerns with AI in healthcare is the lack of transparency in AI-driven decisions. This so-called “black box” problem, where the AI provides a decision or diagnosis without clear explanation, is a significant hurdle. In healthcare, where trust and understanding are paramount, it’s crucial for doctors to comprehend why an AI system is recommending a particular treatment or diagnosis. Without this transparency, it’s challenging for healthcare professionals to trust the AI’s decisions or explain them to patients.
Solution: Enter model interpretability techniques, which are designed to make AI decisions more transparent. These techniques help doctors understand the factors influencing the AI’s recommendations, such as highlighting specific medical images or patient data that triggered a particular diagnosis. For example, some AI models now provide visual explanations of how they arrived at their conclusions, making it easier for physicians to cross-check the AI’s findings with their expertise.
Regulatory Frameworks: Emerging Standards and Guidelines
As AI becomes more integrated into healthcare, there’s a growing need for regulatory standards to ensure these technologies are safe, effective, and used responsibly. Without clear guidelines, there’s a risk that AI could be deployed in ways that don’t fully account for patient safety, data privacy, or ethical considerations. Fortunately, regulators are starting to address these concerns by developing frameworks to guide the development and use of AI in healthcare.
Example: The FDA’s Software as a Medical Device (SaMD) guidelines are a prime example of how regulatory bodies are stepping in. These guidelines provide a clear framework for developing, testing, and approving AI-driven software that functions as medical devices. By treating AI as a medical device, the FDA ensures that AI solutions go through the same rigorous approval process as traditional medical devices, ensuring they meet high standards of safety and effectiveness before being used in clinical settings.
Healthcare AI Readiness Assessment
Is your healthcare organization ready for Generative AI? Evaluate your readiness with our checklist. Here’s a checklist to consider when integrating AI into healthcare. Each of these points is critical for ensuring that AI is used safely, effectively, and ethically:
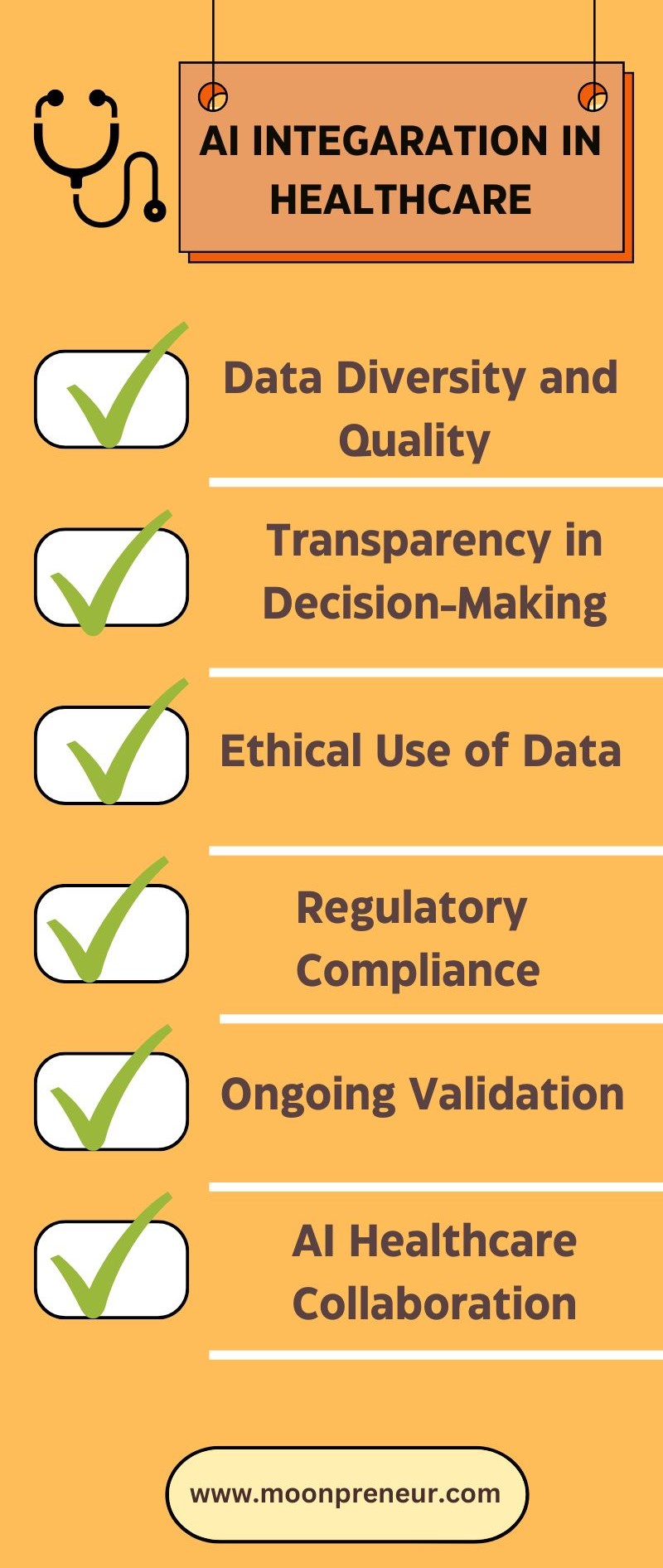
1. Data Diversity and Quality: Embrace the potential of AI by ensuring the system is trained on a broad, representative dataset. This will not only prevent bias but also ensure that the AI works effectively across diverse populations, leading to more accurate and equitable healthcare solutions.
2. Transparency in Decision-Making: AI decisions shouldn’t be a “black box.” It’s crucial to ensure mechanisms are in place to explain how the AI arrives at its conclusions. This transparency will build trust with both healthcare providers and patients, reassuring them that AI is a reliable assistant, not a mysterious force.
3. Ethical Use of Data: Always prioritize patient privacy and ethical data use. AI systems should comply with all relevant privacy laws (like HIPAA) and ensure that patient data is handled responsibly.
4. Regulatory Compliance: Stay up-to-date with the latest regulatory frameworks, such as the FDA’s Software as a Medical Device (SaMD) guidelines. This helps ensure that AI-driven tools are safe, effective, and meet legal standards before being implemented in a clinical setting. Failure to comply with these regulations can lead to legal issues and compromise patient safety.
5. Ongoing Monitoring and Validation: AI systems aren’t “set it and forget it” tools. It’s essential to regularly monitor and validate AI models to ensure they’re performing as expected and not drifting away from accurate predictions over time. This continuous improvement is key to maintaining the reliability and effectiveness of AI in healthcare.
6. Collaboration Between AI and Healthcare Professionals: AI should be seen as a tool to assist healthcare providers, not replace them. For instance, AI can help in diagnosing diseases, predicting patient outcomes, and personalizing treatment plans. Foster a collaborative environment where doctors can combine AI insights with their expertise to make the best possible decisions for patients.
Myths vs Facts about AI in Healthcare
Myth | Fact |
---|---|
AI will make healthcare professionals obsolete. | AI is designed to support and enhance the work of healthcare professionals, not replace them. AI assists doctors by analyzing data quickly, providing recommendations, and flagging areas of concern, but human judgment and expertise remain essential in patient care. |
AI makes perfect, unbiased decisions. | AI systems can inherit biases from the data they are trained on. If the training data is biased, the AI may produce biased outcomes. Ensuring diverse and representative datasets is critical to mitigating this issue, but AI is not immune to the same flaws that affect human decision-making. |
AI will work perfectly right out of the box. | AI systems need ongoing training, validation, and updates to remain accurate and effective. They require continuous input and refinement, especially as new medical knowledge and patient data become available. Healthcare professionals need to regularly monitor and fine-tune these systems to avoid errors or "drift" in performance. |
AI can completely replace clinical trials. | While AI can speed up drug discovery and simulate some trial outcomes, it cannot entirely replace human clinical trials. AI can predict potential treatment responses and generate synthetic data for simulations, but real-world trials are still necessary to ensure safety and efficacy in humans. |
AI systems are self-sufficient. | AI systems rely on healthcare providers for context and accurate input. Even the most advanced AI systems cannot work in isolation—they need data, oversight, and collaboration with doctors, nurses, and other medical staff to be truly effective. |
AI can predict everything perfectly. | AI is powerful, but it has limitations. It can analyze large datasets and identify trends, but it is not foolproof. Factors like incomplete data, patient variability, and unpredictable circumstances can affect AI’s accuracy, meaning human oversight is still critical in final decision-making. |
Patient Perspective: Benefits and Concerns
BENEFITS:
- Enhanced Accuracy: AI’s ability to analyze vast amounts of medical data quickly leads to more accurate diagnoses and treatment plans. This accuracy can instill confidence in healthcare professionals, ensuring they make the best decisions for their patients.
- Personalized Care: AI allows for tailored treatment approaches based on individual health data, leading to more effective therapies that consider a patient’s unique circumstances.
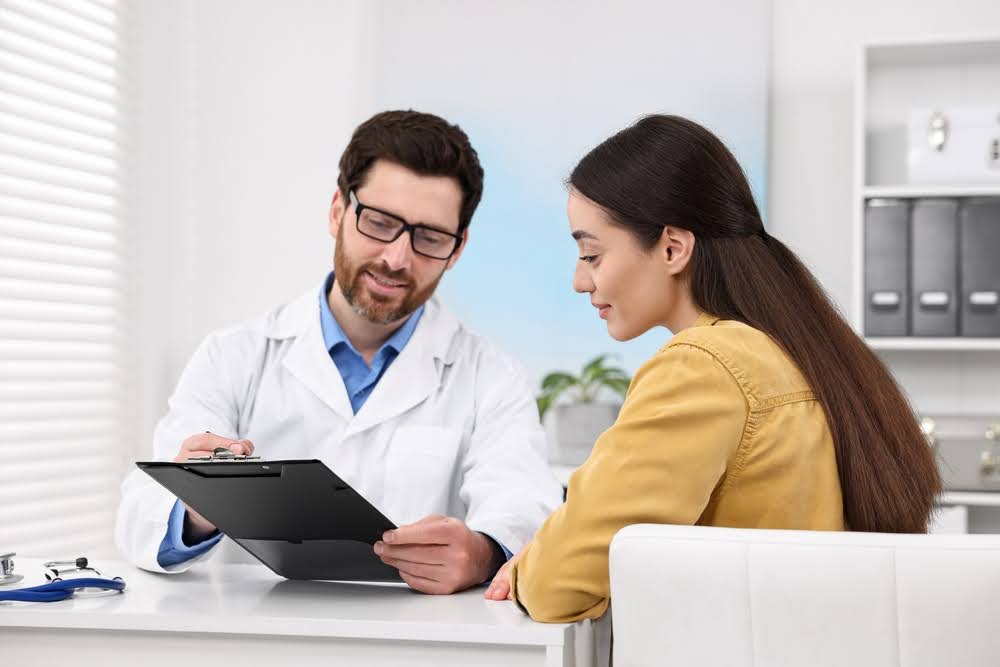
”Generative AI: Empowering Patients with Improved Health Outcomes.”
- Faster Diagnosis: With AI tools aiding in analysis, patients can expect a faster diagnosis, reducing the waiting time for test results and potential treatments. This speed can provide reassurance and reduce anxiety, allowing for quicker access to necessary care.
- Empowered Engagement: AI-driven apps and tools are not just about providing information. They empower patients with personalized health data, reminders, and support, encouraging them to take a more active and informed role in their healthcare.
CONCERNS:
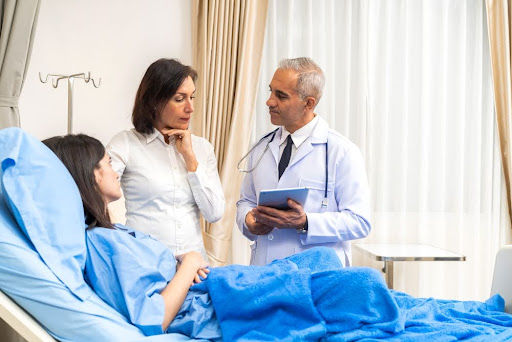
- Data Privacy: Many patients worry about how their personal health data is being used and shared, fearing breaches of privacy and unauthorized access.
- Lack of Transparency: The complexity of AI algorithms can make it difficult for patients to understand how decisions are made, leading to concerns about the reliability of AI-driven recommendations.
- Dependency on Technology: Some patients express apprehension about over-relying on AI, fearing that it may undermine the human touch in healthcare or lead to less personalized care from clinicians.
- Bias and Inequality: Patients may be concerned that AI systems could perpetuate existing biases in healthcare, leading to unequal treatment based on factors like race, gender, or socioeconomic status.
These perspectives highlight the impacts of AI considering both the potential benefits and concerns that patients have regarding AI in healthcare, ensuring that their voices are part of the conversation as technology evolves.
Conclusion
In conclusion, Generative AI in healthcare is not just a technological advancement; it represents a paradigm shift in how we approach diagnosis, treatment, and patient engagement. By enhancing accuracy, personalizing care, and streamlining processes, generative AI is transforming healthcare. As the healthcare industry continues to evolve, Generative AI has the potential to transform healthcare delivery for the better. However, as we embrace this innovation, it’s essential to address concerns about data privacy, transparency, and bias to ensure that all patients benefit equitably. As we continue to explore the possibilities of generative AI, we are paving the way for a future where healthcare is not only more efficient but also more compassionate and inclusive.
Moonpreneur is on a mission to disrupt traditional education and future-proof the next generation with holistic learning solutions. Its Innovator Program is building tomorrow’s workforce by training students in AI/ML, Robotics, Coding, IoT, and Apps, enabling entrepreneurship through experiential learning.
Generative AI in healthcare reminds me of how educational technology has transformed learning experiences for kids. Just like AI can personalize healthcare, ed-tech platforms now tailor learning paths to fit each child’s unique abilities. My child’s app uses data to offer individualized learning suggestions, similar to how AI here provides personalized patient care. It’s fascinating to see AI play a role in making experiences—whether learning or healthcare—more effective and tailored.
I appreciate the advancements generative AI brings to healthcare, but as a parent, I have some concerns. Just like with kids’ educational apps, which sometimes focus too heavily on data-driven suggestions, healthcare AI may risk overlooking the human side of care. It would be beneficial to incorporate more patient-centered elements that ensure a holistic view of health—not just what the data says. For instance, involving patient feedback loops, much like we see in user-friendly learning apps, could help refine AI’s approach. Such steps might build more trust and comfort among patients using AI-driven services.