In the ever-evolving landscape of artificial intelligence (AI), optimization plays a pivotal role in enhancing model performance and efficiency. Among the various techniques that have emerged, prompt tuning has gained significant attention. This blog will explore the concept of prompt tuning, its mechanisms, advantages, applications, and challenges, providing you with a comprehensive understanding of this innovative approach.
Understanding Prompt Tuning
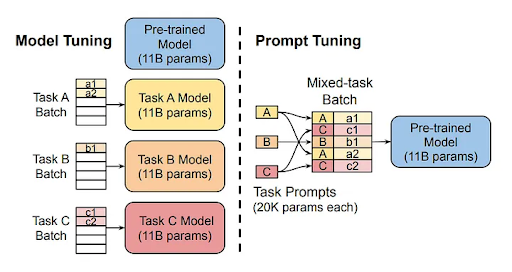
Image Source: https://arxiv.org/abs/2104.08691
Prompt tuning, a highly efficient technique, is used to optimize language models by fine-tuning the prompts or input queries that guide the model’s responses. Unlike traditional fine-tuning, which involves retraining the entire model, prompt tuning focuses solely on these specific prompts. The key objective of prompt tuning is to improve a model’s performance on particular tasks without the need for extensive retraining, making it a practical and attractive option for developers.
The Mechanism of Prompt Tuning
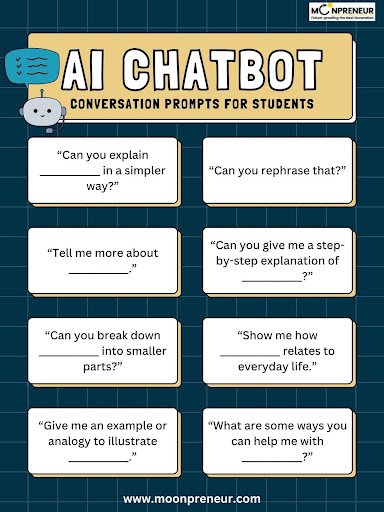
Understanding how prompt tuning works is crucial to appreciating its impact on AI optimization. The process involves crafting effective prompts that guide the model’s behavior. By tweaking the wording, structure, or context of these prompts, developers can significantly influence the model’s output.
One notable aspect of prompt tuning is its reliance on prompt templates. These templates serve as foundational structures that can be modified to fit specific tasks. For instance, if a model is designed for question-answering, a well-structured prompt can lead the model to provide more accurate and contextually relevant answers. Prompt templates play a crucial role in guiding the model’s responses.
Furthermore, prompt tuning is not to be confused with prompt engineering, which often involves manual adjustments to prompts based on trial and error. Prompt tuning, on the other hand, is a systematic approach to optimizing prompts for better performance across various tasks, providing a reliable method for AI developers.
Recommended Reading: AI Strategist: Guiding Businesses Through the AI Revolution
Advantages of Prompt Tuning
There are several compelling advantages to using prompt tuning in AI optimization:
- Efficiency in Training: One of the primary benefits of prompt tuning is its efficiency. By focusing on prompts instead of the entire model, developers can achieve faster training times. This is especially valuable when dealing with large language models that require significant resources for full retraining.
- Reduced Computational Costs: Alongside faster training, prompt tuning also leads to lower computational costs. Since only the prompts are adjusted, there is less demand for hardware, making it more accessible for smaller teams or organizations with limited resources.
- Enhanced Performance on Specific Tasks: Prompt tuning allows for targeted improvements in model performance. By optimizing prompts for specific tasks, developers can achieve better accuracy and relevance, making AI systems more effective in real-world applications.
Applications of Prompt Tuning
Prompt tuning has vast and varied real-world applications. Industries such as customer service, healthcare, and finance are beginning to leverage this technique to enhance their AI capabilities.
Case Studies of Prompt Tuning in Action:
Customer Support: OpenAI’s ChatGPT
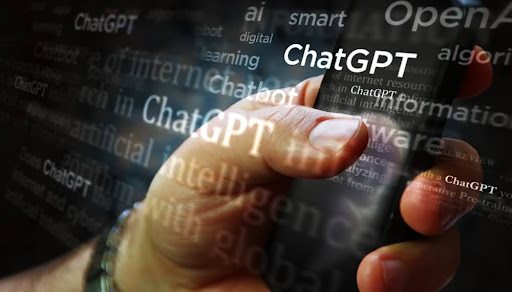
Background: OpenAI’s ChatGPT, a conversational AI, was designed to provide engaging dialogue and text generation across various applications. Its adaptability has seen it being used in customer support roles across industries, automating responses and improving customer service efficiency.
Application: By integrating ChatGPT with customer service platforms (e.g., Zendesk), companies fine-tuned prompts to handle customer queries efficiently. ChatGPT excels in drafting responses, analyzing customer sentiment, and generating support scripts for agents. The AI model’s flexibility allows it to handle diverse inquiries, from FAQs to complex issues, ensuring prompt responses with limited human intervention.
Outcomes :
- Efficiency Gains: Businesses using AI-powered tools like ChatGPT have reported substantial improvements in agent productivity, with some experiencing up to a 30% increase.
- Customer Satisfaction: Automating initial customer interactions has led to an increase in customer satisfaction by up to 50%. This is primarily due to faster response times and more personalized interactions, which are made possible by prompt tuning.
- Reduced Response Time: The average handling time for customer inquiries decreased by 20%, leading to quicker resolution of support tickets
Content Generation: Jasper AI
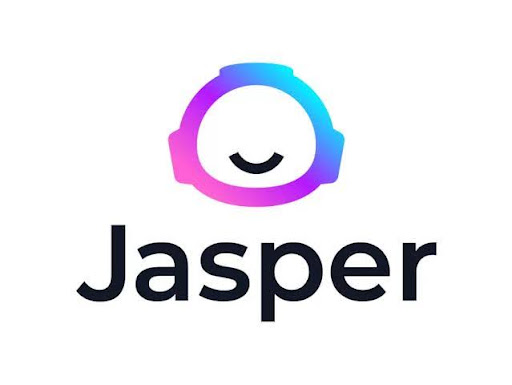
Background: Jasper AI, a leading content creation tool, empowers marketers, content creators, and businesses to efficiently generate high-quality articles, social media posts, and marketing content. By harnessing GPT-based technology, Jasper can adapt to various tones and styles, providing a versatile and powerful solution for content needs.
Application: Jasper uses prompt tuning, a process of refining the input given to the AI model to produce more accurate and relevant outputs, to optimize content generation for different target audiences. Businesses have used Jasper to write blogs, ad copy, and social media content tailored to specific campaigns and audiences, ensuring better alignment with marketing goals. The tool’s capacity to rapidly produce content has been a game-changer in reducing the workload for marketing teams.
Outcomes:
- Increased Content Output: Users reported a 70% increase in content output compared to manual writing processes.
- Time Efficiency: Jasper reduces content creation time by over 50%, allowing marketers to focus on strategy rather than drafting.
- Engagement Improvement: Companies using Jasper have experienced a 200% increase in click-through rates for AI-generated ads and higher engagement for blog and social media posts.
Healthcare: Cohere
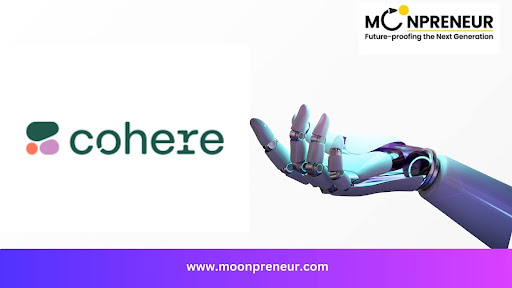
Recommended Reading: Generative AI in Healthcare: Bridging the Gap Between Data and Diagnosis
Background: Cohere specializes in using AI to streamline healthcare operations, such as claims management and data analysis. This enables healthcare providers to focus more on patient care rather than administrative tasks. Cohere’s AI models, powered by natural language processing (NLP), are designed to understand and process complex healthcare data, making it a valuable tool for improving operational efficiency in the healthcare sector.
Application: Cohere uses natural language processing (NLP) to process patient data, assist in claims management, and diagnose through pattern analysis in patient records. By optimizing the language models used to analyze healthcare data, Cohere helps reduce errors in patient information handling and speeds up the time taken for administrative tasks.
Outcomes:
- Cost Reduction: Automating healthcare workflows with AI has led to a 40% reduction in operational costs, primarily by lowering administrative workload and improving process efficiency.
- Error Reduction: By streamlining data processing, error rates in claims and documentation have been reduced by 25%, leading to better patient outcomes and more accurate medical records.
- Improved Patient Care: With more time focused on patient care, healthcare providers using Cohere’s AI models report enhanced diagnostic accuracy and quicker decision-making processes.
Legal Analysis: Casetext
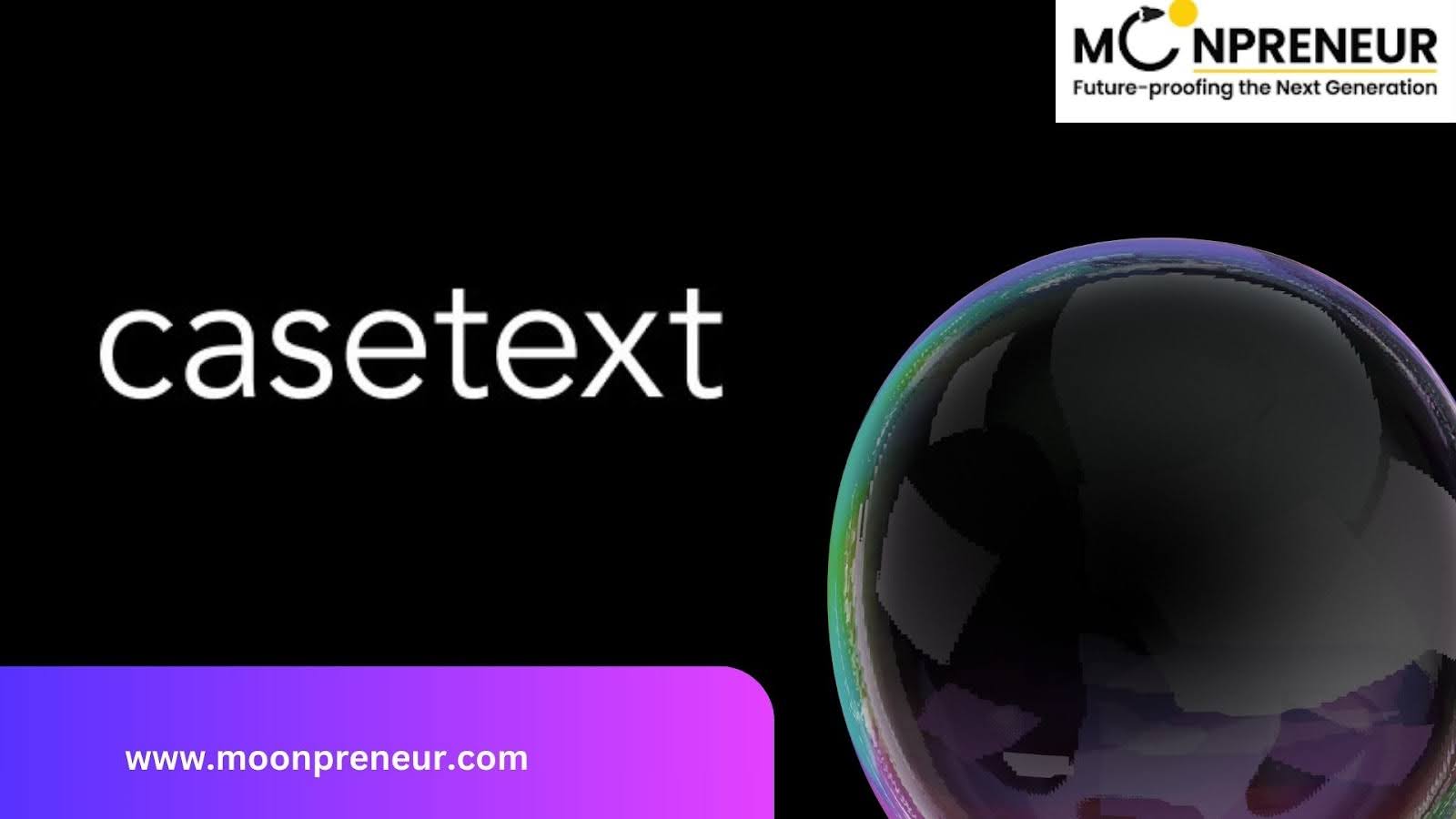
Recommended Reading: A Kid’s Guide to Prompt Engineering: Teaching AI to Think
Background: Casetext offers AI-driven legal research tools designed to assist lawyers in parsing case law, statutes, and legal documents quickly. Casetext’s AI, powered by their CoCounsel platform, uses NLP to simplify legal document review and analysis. Through prompt tuning, Casetext enhances its AI model’s ability to understand and summarize complex legal documents, providing insights in a fraction of the time compared to traditional methods.
Application: Through prompt tuning, Casetext enhances its AI model’s ability to understand and summarize complex legal documents. Lawyers use Casetext to perform research, draft arguments, and analyze legal contracts. By improving prompt clarity and structure, the AI delivers more accurate and insightful results, reducing the time required for document review.
Outcomes:
- Time Savings: Firms using Casetext have reported a 24% reduction in legal research time, which allows attorneys to focus more on strategy and case preparation.
- Accuracy: Legal analysis accuracy improved by 30%, giving lawyers more confidence in the AI’s ability to provide relevant insights.
- Document Review Efficiency: The platform cuts down contract and document review time by 50%, making it easier for legal professionals to meet tight deadlines.
Educational Tools: Tailoring Prompts for Personalized Learning
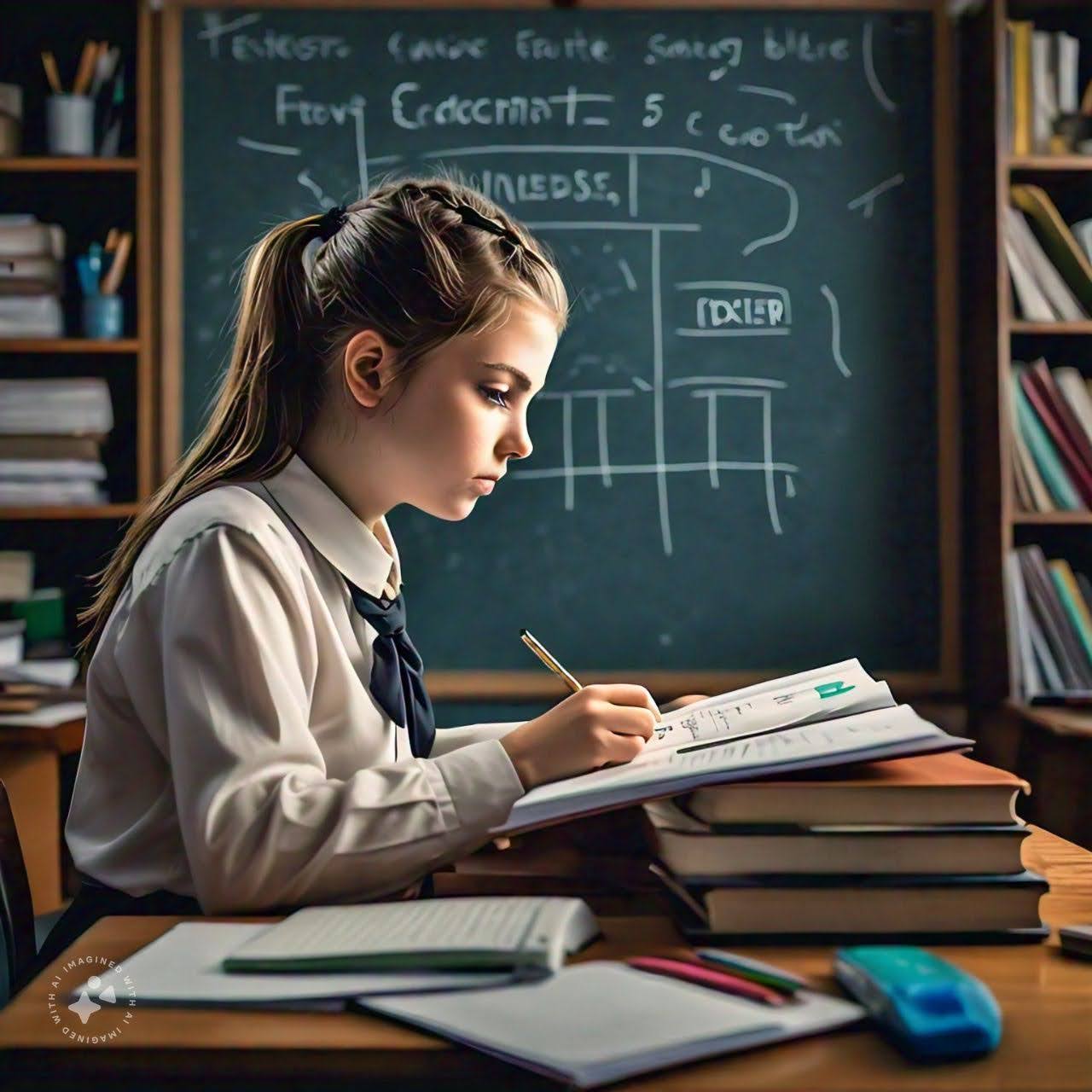
Background: AI is increasingly being used in educational tools to provide customized learning experiences. By leveraging AI models, educational platforms can adapt to each student’s learning style and pace, making learning more efficient and engaging.
Did you Read ?
Application: In educational settings, prompt tuning is used to tailor AI systems to individual learners. For example, AI-driven platforms like Squirrel AI and DreamBox Learning apply prompt tuning to generate personalized problem sets, explanations, and study guides based on student progress and performance. The prompts are optimized to challenge students appropriately, ensuring that they receive personalized feedback and content that aligns with their learning needs.
Outcomes:
- Improved Student Engagement: Personalized learning tools using prompt tuning have been shown to increase student engagement by 20%, as lessons are more relevant and adapted to individual skill levels.
- Learning Efficiency: Schools using AI-powered educational platforms reported a 30% improvement in learning efficiency, with students achieving mastery of concepts faster than in traditional settings.
- Enhanced Retention: Adaptive AI learning systems also contribute to better knowledge retention, with an average increase of 15% in student retention rates, thanks to customized learning paths.
Step-by-Step Guide to Implementing Prompt Tuning
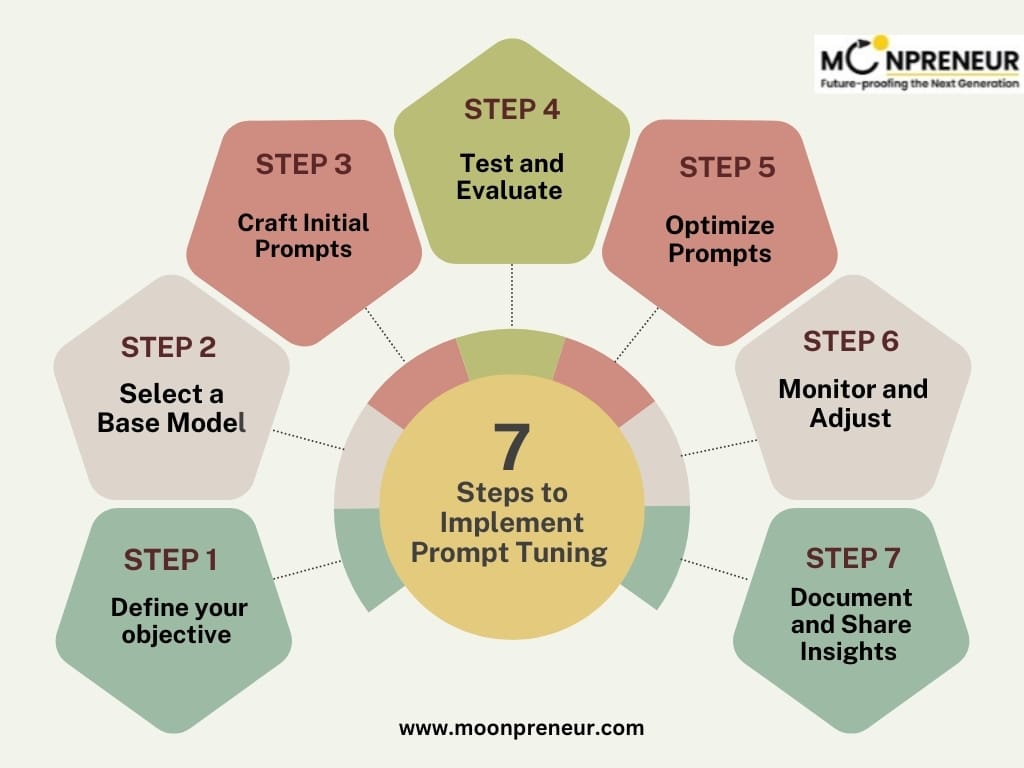
Identify the specific task you want to optimize, such as customer support or content generation.
Pro Tip: Define clear objectives using the SMART criteria (Specific, Measurable, Achievable, Relevant, and Time-bound).
Common Pitfall: Avoid vague objectives; they can lead to ineffective tuning.
Choose a pre-trained language model that aligns with your task.
Popular Options: Hugging Face Transformers, OpenAI’s API.
Create a set of initial prompts focused on clarity and context to ensure practical guidance for the model.
Example: Instead of asking, “What is AI?”, try “Can you explain AI in simple terms for a beginner?”
Run your model with the initial prompts. Evaluate performance using relevant metrics.
Metrics to Consider: Accuracy, relevance, user satisfaction.
Iterate on your prompts based on evaluation results. Modify wording, structure, or context to enhance performance.
Tip: Keep a journal of prompt iterations to track changes and outcomes.
Common Mistake: Avoid making multiple changes at once; it complicates analysis.
Continuously monitor the model’s performance after deployment, making adjustments as new data and use cases emerge.
Tools: Analytics tools are used to monitor user interactions.
Keep a record of your process and findings. Sharing insights with your team can lead to collaborative improvements and innovations.
Challenges and Solutions
Despite the many benefits of prompt tuning, it does come with its own set of challenges. Here are some common issues developers and researchers face with their respective solutions:
Challenges | Solutions |
---|---|
Dependence on Quality Data |
|
Limited Generalizability |
|
Complexity in Crafting Effective Prompts |
|
Tools and Frameworks for Prompt Tuning
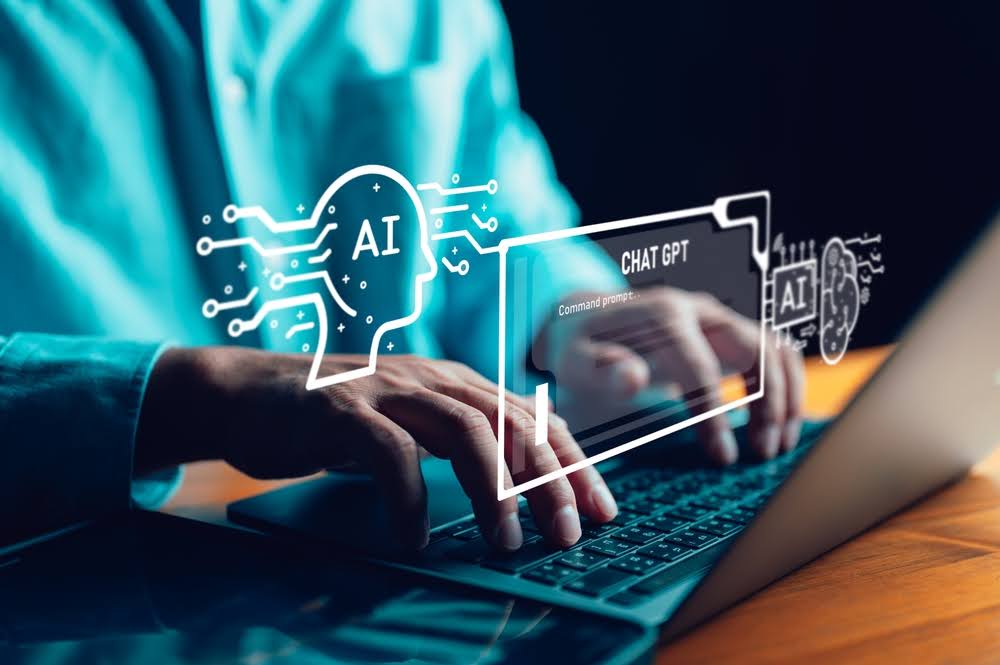
If you’re ready to take control and dive into prompt tuning, you’re in luck! Several tools and frameworks can empower you to simplify the process and make it more accessible. Here are some popular options that put the power in your hands:
1. Hugging Face Transformers
- Overview: Hugging Face Transformers is a widely used library that provides pre-trained models and a user-friendly interface for implementing prompt tuning techniques.
- Features: The library’s user-friendly interface and comprehensive documentation make it a comfortable choice for developers, as it’s easier to fine-tune prompts for specific tasks. It also includes tools for model evaluation and deployment, enhancing usability across applications.
- Benefits: Developers can tap into a supportive community and leverage the extensive model hub to experiment with different architectures and approaches to prompt tuning, knowing that they are not alone in their journey.
2. OpenAI’s API
- Overview: OpenAI’s API provides access to powerful language models that can be fine-tuned using prompt tuning strategies.
- Features: The API allows developers to integrate advanced natural language processing capabilities into their applications without needing extensive machine learning expertise. OpenAI provides a set of tools for prompt engineering, making it easier to craft effective prompts for various tasks.
- Benefits: With robust models like GPT-3, developers can efficiently achieve high-quality text generation, summarization, and other NLP tasks.
3. Prompt Toolkit
- Overview: The Prompt Toolkit is a specialized tool designed to help developers create and manage prompts effectively.
- Features: It provides a set of utilities for building interactive command-line applications, allowing developers to customize prompts, handle input, and manage output more seamlessly.
- Benefits: This toolkit is particularly useful for applications that require dynamic user interactions and can improve the overall user experience in prompt-driven environments.
When selecting a tool, consider your project’s needs, including the specific tasks you’re aiming to optimize and the resources available to you.
Conclusion
In conclusion, prompt tuning represents a significant advancement in AI optimization, providing developers with a powerful tool to enhance model performance efficiently. By understanding the mechanisms, advantages, applications, and challenges of prompt tuning, you can leverage this technique to improve your AI systems effectively.
As the field of artificial intelligence continues to evolve, staying informed about innovative techniques like prompt tuning will be crucial for those looking to maintain a competitive edge. We encourage you to explore prompt tuning further and consider its potential to elevate your AI projects.
Moonpreneur is on a mission to disrupt traditional education and future-proof the next generation with holistic learning solutions. Its Innovator Program is building tomorrow’s workforce by training students in AI/ML, Robotics, Coding, IoT, and Apps, enabling entrepreneurship through experiential learning.
Prompt tuning seems like an intriguing approach to refining AI model performance, especially in optimizing for specific tasks. Considering its potential, how do you see this technique balancing resource efficiency versus the complexity of implementation for smaller organizations? It would be interesting to explore how this compares with other fine-tuning methods, particularly for non-expert users aiming to implement AI solutions.
Prompt tuning seems like a promising method for optimizing AI without extensive resource demands. I’m curious about its limitations—are there scenarios where traditional fine-tuning might still outperform this technique? Also, how adaptable is prompt tuning for tasks outside of NLP, such as vision-based AI systems?
Great question! Prompt tuning excels in efficiency for task-specific NLP applications, but it has its limitations. For tasks requiring significant generalization or flexibility, traditional fine-tuning may perform better, as it adapts the model’s deeper parameters. While prompt tuning is primarily used in NLP, its application to other domains like vision-based AI is still emerging. Techniques like soft prompting are being explored for vision tasks, but they currently face challenges due to differences in how visual data is structured compared to text. In this blog, a great example of ‘Cohere’ is provided, demonstrating how prompt tuning is being adapted for various fields.